Fraud has plagued the lending industry for many years. Fraudulent behavior in lending can take many forms, including identity theft, loan stacking, and money laundering. To combat these issues, lenders increasingly turn to artificial intelligence (AI) for fraud detection.
AI has the potential to transform fraud detection in lending by enabling lenders to analyze vast amounts of data and detect patterns that may be indicative of fraudulent activity.
In this blog, we will explore seven use cases of AI for fraud detection in lending and how they can be leveraged to mitigate the risk of fraud and protect lenders and borrowers from financial losses.
How Does AI in Fraud Detection in Lending Works?
AI-based fraud detection systems typically use machine learning algorithms to analyze historical data and identify patterns that may indicate fraudulent activities. These algorithms are trained using Large datasets of historical transaction data, allowing them to recognize patterns indicative of fraud. Machine learning algorithms can be supervised or unsupervised, depending on the level of human involvement required in the training process.
Supervised machine learning algorithms are trained using labeled data, which means that the algorithm is provided with examples of fraudulent and non-fraudulent activities. The algorithm learns to recognize patterns in the labeled data and can then apply this knowledge to detect fraud in real-time.
On the other hand, unsupervised machine learning algorithms are trained using unlabeled data. The algorithm needs to be provided with examples of fraudulent or non-fraudulent activities. Instead, it learns to identify patterns and anomalies in the data independently. Unsupervised machine learning algorithms are particularly useful for detecting new and previously unknown types of fraud.
In addition to machine learning algorithms, AI for fraud detection in lending may also involve using natural language processing (NLP), which enables the system to analyze unstructured data such as emails and chat logs. NLP can identify specific keywords and phrases that may indicate fraudulent activities, enabling the system to detect potential fraud in real-time.
Top 11 use cases of AI for fraud detection in Lending
1. Automated Document Processing
One of the most time-consuming aspects of the lending process is document processing. Traditional lenders require extensive documentation from borrowers, including bank statements, tax returns, and proof of income. This can create a significant bottleneck in the lending process, slowing approvals and increasing the likelihood of errors and fraud.
AI can automate document processing, making it faster and more accurate. Using machine learning algorithms to analyze documents, lenders can quickly identify fraudulent information or discrepancies, reducing the risk of fraud while also improving the speed and efficiency of the lending process.
2. Identity Verification
One of the most common forms of lending fraud is identity theft, where bad actors use stolen identities to secure loans or credit. AI can detect and prevent identity theft by verifying the identity of borrowers using biometric data, facial recognition, and other advanced techniques. By leveraging AI for identity verification, lenders can reduce the risk of fraudulent applications while also improving the user experience for legitimate borrowers.
3. Payment Fraud Detection Models
AI can be used to develop payment fraud detection models that are capable of analyzing vast amounts of data to identify patterns and anomalies that may indicate fraud in Lending. These models can be trained using historical data to identify common payment fraud patterns, such as loan stacking or fake identities. By continuously monitoring loan applications and identifying fraudulent activity in real-time, lenders can significantly reduce the risk of fraud and improve the overall security of the lending process.
4. Behavioral Analytics
AI can be used to analyze borrower behavior and detect patterns that may indicate fraud. By analyzing user navigation, click patterns, and time spent on each page, lenders can identify suspicious activity that may indicate a fraudulent application. By leveraging behavioral analytics, lenders can identify and prevent fraud in real-time, reducing losses and improving the overall security of the lending process.
5. Natural Language Processing
Lending applications often include free-form text fields where borrowers can provide additional information or context. Natural language processing (NLP) can be used to analyze these fields, identifying keywords and phrases that may indicate fraud or other suspicious activity. By analyzing this data in real-time, lenders can quickly identify and prevent fraudulent applications, reducing losses and improving the overall security of the lending process.
6. Eliminating the flow problem
The flow problem in lending refers to the slow and cumbersome lending process that can result in delays and inefficiencies for borrowers. AI can streamline lending processes, making them faster, more efficient, and less subjective. By automating manual processes and using data-driven algorithms to evaluate borrowers, AI-powered lending platforms can significantly reduce the time and effort required to secure financing.
For example, AI algorithms can analyze various data sources to evaluate a borrower’s creditworthiness, including financial data, credit reports, and social media profiles. This allows lenders to make faster and more informed lending decisions, reducing the time required to approve a loan.
7. Fraudulent Network Analysis
Fraudulent network analysis involves analyzing relationships and connections between individuals and organizations to identify potential fraud. Using machine learning algorithms to analyze data from social networks, public records, and other sources, lenders can identify links between borrowers and other entities that may indicate fraudulent activity.
For example, fraudulent network analysis can be used to identify groups of individuals submitting fraudulent applications together or using the same fraudulent identities to secure multiple loans. By identifying these networks of fraud, lenders can take proactive steps to prevent future losses and improve the overall security of the lending process.
8. Predictive Analytics
AI can perform predictive analytics, which involves using historical data to predict future events. In lending, predictive analytics can be used to identify potential fraudulent activity before it occurs. By analyzing data from multiple sources, including credit scores, financial records, and transaction histories, AI algorithms can identify patterns and trends that may indicate fraudulent activity. This allows lenders to take proactive steps to prevent fraud before it occurs.
9. Anomaly Detection
Anomaly detection involves using machine learning algorithms to identify unusual patterns or behavior that may indicate fraudulent activity. For example, AI algorithms can detect unusual changes in account balances, transaction volumes, or customer behavior. By identifying these anomalies, lenders can investigate potential fraud and take appropriate action to prevent losses.
10. Voice and Face Recognition
AI-powered voice and face recognition technologies can be used to verify the identity of borrowers. By using biometric data to confirm a borrower’s identity, lenders can significantly reduce the risk of identity theft and other types of fraud. Voice and face recognition can be particularly useful for remote lending scenarios, where borrowers may not b
e physically present.
11. Real-time Monitoring
Real-time monitoring involves using AI algorithms to monitor lending activity in real-time. By analyzing transaction data and other sources of information, AI algorithms can identify potential fraud as it occurs, allowing lenders to take immediate action to prevent losses. Real-time monitoring can be particularly useful for high-risk lending scenarios, where fraudulent activity is more likely to occur.
Conclusion
AI has emerged as a powerful tool for detecting and preventing fraud in lending. Using AI algorithms and machine learning techniques, lenders can analyze vast amounts of data to identify potential fraudulent activity and take proactive steps to prevent it.
As the lending industry continues to evolve, we can expect to see even more advanced AI-based techniques emerging for fraud detection. These innovations will make it easier for lenders to detect and prevent fraud, improving the overall security and reliability of the lending process. By adopting AI for fraud detection, lenders can build trust with borrowers, protect their investments, and contribute to the growth and development of the lending industry
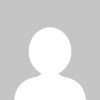
Leave a Reply