Invoices are the lifeblood of any business, containing a wealth of crucial information like customer and vendor details, order specifics, pricing, and taxes. The catch? Extracting data from invoices and matching this data from invoices to other documents can be … Read More
Knowledge Graph
Knowledge Graph
The Knowledge Graph is a valuable tool in Artificial Intelligence for organizing and understanding vast amounts of data. Through machine learning, it transforms complex data into comprehensible knowledge graphs, facilitating communication between business leaders and customers. Widely used in applications like chatbots, recommended systems, and internet searches, knowledge graphs play a crucial role in industries such as eCommerce and banking, aiding in product analysis and customer assistance.
What is Knowledge Graph?
The Knowledge Graph is a valuable tool in Artificial Intelligence for organizing and understanding vast amounts of data. Through machine learning, it transforms complex data into comprehensible knowledge graphs, facilitating communication between business leaders and customers. Widely used in applications like chatbots, recommended systems, and internet searches, knowledge graphs play a crucial role in industries such as eCommerce and banking, aiding in product analysis and customer assistance.
Benefits - Make Better Decisions with Data

Enhanced context
Knowledge graphs help code hierarchy, metadata, unstructured and complex data and various properties.
It also helps query the integrated data, uncover patterns and discover integral connections to get clear and descriptive results.

Future-proof data model
There is always going to be a steady flow of data, such as a new external data stream, a new data source needed for the next version, a new acquisition with its data chaos, etc.
You can use an extensible data model to easily merge
the new sources while preserving the
original schema and
metadata, thus becoming a future-proof data model.

Designed for what's important to your data
Knowledge graphs represent real-world entities and help in understanding complex relationships.
The knowledge graph can maintain multiple perspectives at the same time.

Save time
Manually deciphering large amounts of data using traditional techniques can be time-consuming and expensive, involving many workforces in related tasks. AI-enabled knowledge graphs can help convert data into understandable material that saves time, effort and money.

High performing graphs
Knowledge graph technology helps provide relevant facts and contextual answers to a particular question, rather than extensive search results that contain many relevant documents and news. A full-fledged knowledge graph can provide your organisation with a solid foundation and fundamental solutions for any intelligent application with this integration, enabling you to get good search results.

Easily layered into existing infrastructure
Virtualisation provides resource-saving access to various data, both locally and in the cloud. Access data without displacing or duplicating the original content, preserving original ownership and governance while avoiding data spikes.

Reveal insights
Knowledge graphs reveal insights, search for information and diligently dig through the stack of documents to find specific phrases, numbers, etc. It compiles a network of “things” and the facts related to those "things," creating a strong point of information, facets and attributes of a business, such as a project, product, employee or skill. Each graph can create relatable databases and be connected to other graphs and relational databases.
Use Cases

Intuitive, Natural Language Search
The knowledge graph helps to construe natural language queries and enables you to understand the terms, units, guidelines and associations that the search represents or implies. Integrating knowledge graphs into strategic plans enables a shift from risk mitigation to proactive risk management, predicting and addressing compliance and operational risks.

Find relevant Content and Information
The knowledge graph, effective with unstructured content, serves as a powerful tool for deciphering and authorizing data in complex environments. Once constructed, it enables the integration of chatbots or user-friendly search interfaces, empowering customers and business users to comprehend charts, graphs, and reports. Enterprises leverage this AI and machine learning technology to extract intricate patterns, simplify data, and make informed decisions.

Reliable Content and Data Governance
In the context of a large government agency with 15,000 datasets, data scientists faced challenges in access and reliability despite the creation of a data dictionary and a centralized platform. Knowledge graphs provided enhanced control, enabling data scientists to search records based on relationships between data items and record movements. This allowed precise control over viewing permissions and tracking record changes. Through knowledge graphs, users now navigate a complex area more effectively, gaining access to data beyond previous dataset limitations.

Predict compliance and Operational Risk
With the help of knowledge graphs, incorporating them into your strategic plan, you can move from proactive risk mitigation to proactive risk management. It helps reduce the risks and proactively reports them to regulatory agencies in case of non-compliance.Converting complex data into knowledge graphs enables enterprises to identify network errors, address theoretical risks, answer complex questions, reuse data, maintain processes with key personnel, and assess the overall impact of each threat.
Blogs
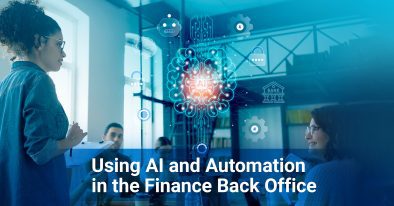
Using AI and Automation in the Finance Back Office
With the advent of Artificial Intelligence (AI), Machine learning (ML), Natural Language Processing(NLP), and Robotic Process Automation (RPA), businesses can streamline workflows, cut costs, and foster sustainable growth amid market challenges. Despite advancements in enterprise resource planning (ERP) systems, a … Read More
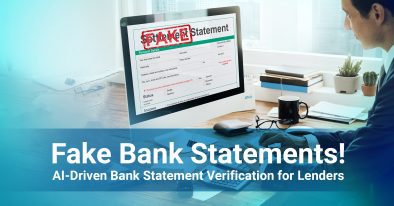
How to Spot Fake Bank Statements
With evolving digital transactions and sophisticated fraud techniques, the need for robust fraud detection mechanisms is equally increasing. One area where this is particularly pertinent is in identifying fake bank statements, a common tool fraudsters use to manipulate financial information. … Read More