Artificial Intelligence programmes are steadily changing our personal and professional lives. You can now apply machine learning to banks, financial services and insurance industries worldwide. The focus is on delivering AI as a service and using a self-developed machine learning platform to solve customer analytics and automation issues. Multiple AI-enabled platforms and algorithms extract deep insights from big data powered by Artificial Intelligence and deep learning, using them to drive decision making and automate client extraction.
Auditors seek to keep up with technology’s rapid adoption and evolution in an increasingly data-driven world, and the change is already being felt. More than ever, auditors and audited entities are using next-generation technology. Audits are always accountable and are often performed to protect the privacy of the information stored in the database. With the widespread use of databases in businesses and the internet, concerns about privacy policies and practices are steadily rising.
AI-based audits enable system administrators to implement a high degree of protection, identify suspicious activity early and take fine-grained security measures. Many companies use a manual audit process to validate and comply with billing and financial information. Enterprises can capture critical content to automate the review process and improve efficiency.
You can automate the auditing process, which is known to-
- Reduce lead time by 19-40%
- Check the validation of all generated statements
- Maintain the original layout during data extraction
- Achieve 100% test coverage of documents
- Understand the important context recorded in the footnote
Why Do Audits Need Automation?
1. Risk Assessment
Automation is necessary to understand risks, variations and inconsistencies within the data. These risks help identify audit needs and plans.
2. Labour-intensive execution
Organisations need auditing done on huge amounts of data for which traditional methods don’t work. AI-automated auditing enables companies to audit these large collections of data.
3.Inaccurate interpretation of data
There are several intricacies to data like graphs, pie charts, diagrams and other forms of statistical data, which was difficult for the conventional auditing methods to comprehend. However, automation is required to decipher such complicated data.
4. False Data Analysis
The verification, integration and validation process of automation is much better than the traditional methods used mainly because of the error/false percentage. It has been observed that the errors in the reporting of old methods are much greater than the automated reports. Data are significantly affected by superscripts, spaces, special characters and untitled text.
5. Use of cognitive Intelligence
AI-automated auditing techniques diligently use natural language generation, natural language processing and machine learning that help mimic human behaviour and perform better than human intelligence, generating accurate results. It encompasses various processes like decision making, visual perception, speech recognition, translation between languages, etc.
6. Manual cross-references
Some statements and company documents contain reference data. Identifying these references and understanding and deriving the information is a time-consuming process.
Considering the flaws of the manual auditing processes and the pros of automated auditing, it is highly recommended that all firms and organisations delve into AI-enabled processes to automate auditing and ensure compliance procedures.
Understand the Audit Workflow Automation of an Automated Audit Platform
Step 1 – Uploading the content– This is where the content is uploaded to the platform.
Step 2 – The document preprocessor works after the preprocessing statement is fully uploaded. Here, the following operations are performed:
- The preprocessor scans the entire document, breaks it down into pages and sections and selects the page that contains the relevant data.
- The preprocessor divides, tacks and trims data to reduce disparities and improve text clarity.
Step 3 – AI segments and identifies images of the extracted data at this stage of the audit workflow automation.
Step 4 – Identify the document section that contains the table structure. It then provides a unique function for extracting data from these tables. This allows you to seamlessly extract data while understanding the relevant context.
Step 5 – This phase uses core AI features such as machine learning, natural language processing and predictive analytics to extract text from a company’s financial statements.
Step 6 – ML enrichment automation understands the context of statements to enhance the raw text.
Step 7 – The NLP-based classification framework categorises the extracted text. This framework is made possible by understanding the context so that you can identify whether it contains financial details related to the data.
Step 8 – NLP skills use custom models for extracting key entities related to statements.
Step 9 – AI implements context data validation and modification algorithms on the data extracted for validation in the audit workflow automation.
Step 10 – Aggregation and Integration – This consolidates the output into one document and ultimately integrates the aggregated data with other applications within the organisation’s ecosystem.
Features of AI-Enabled Audit Process
1. Layout Conservation
This feature helps understand raw data, preserves the natural layout and helps analyse unstructured content. It helps in comprehending different kinds of layouts and the data it contains, no matter its complexity.
2. Processing of Variation Elements
The AI-based audit processes are well-equipped with the intelligence of ML, NLP, IDP and predictive analysis, which helps to decipher complicated data like images, text, diagrams, graphs, etc. Image recognition is a key feature that helps comprehend all kinds of pictures and video graphics.
3. Footnote Extraction
The traditional means of auditing have not been capable enough to understand the complexities of the footnotes, which carry vital information.
Extraction of footnotes includes:
- Superscript analysis to help recognise, classify and extract data from superscripts to generate insights.
- If a statement has a section without a title or a heading, and the manual audit mechanism does not recognise such a section of the statement, the core AI feature will identify and extract such data.
- AI-enabled platforms can understand, map, authenticate and identify references across different sources, platforms and formats.
- The automated inspection helps you understand, analyse and extract information from different fonts and styles.
- While traditional auditing methods often take time and effort, AI-enabled intelligent data acquisition platforms automate the process and reduce the turn-around time to a large extent.
Conclusion
The AI-enabled platform is armed with principal AI components such as computer vision, natural language processing, machine learning and predictive analytics. AI and machine learning examine records and train the platform. The automated platform of your choice can understand, classify, isolate, extract and validate data from various formats and layouts. This speeds up the process of verifying large numbers of corporate statements, reveals authentic, unbiased results and helps maintain and guarantee compliance throughout the year.
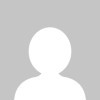
Leave a Reply