In recent times, financial services involve extensive unstructured data sets that are constantly increasing in complexity and variety. Given this ambiguity, it becomes challenging for businesses to manage this unstructured data in a database format. Also, the complex nature of this unstructured data can remain hidden in various places. Your employees might have to spend a lot of their valuable time finding relevant data from business documents, CRM (Customer Relationship Management) systems, or other data sets.
Further, manual data segregation is usually prone to several errors and is expensive for businesses. This is where financial data automation comes into the picture to help you overcome these challenges of unstructured content. Keep reading the article below to learn more about the role of financial automation in streamlining unstructured data in financial services.
Understanding the Role of Unstructured Data in Financial Data Automation
Unstructured data in recent times has played a considerable role in the financial service domain. This unstructured data usually assists in delivering insight into changing client requirements. It allows the banking sector and financial institutions to remain up-to-date with the contemporary trends to stay ahead of their opponents.
However, structured and unstructured data fields related to the finance and banking sector remain independent and have automated functions that predominantly focus on structured database files. This increased emphasis on structured data created considerable challenges for unstructured data types, such as complicated financial services management. Hence, it becomes imperative for financial institutions to better address large quantities of unstructured data to let them succeed in the online domain.
Similarly, financial institutions must quickly transform their documents into actionable data, gain valuable industry insight, and understand where banking financial data automation can offer the greatest value.
Ways to Overcome the Difficulties of Unstructured Content in Financial Services
Access to real-time financial information is necessary for good business decisions. However, conventional financial institutions’ operations need a substantial turnaround time to generate NLP for financial documents and reports. Usually, this results in the untimely delivery of predictive insights.
Here are some ways to help you overcome the challenges of unstructured data in financial services and take a step towards financial data automation in banking.
Determine the business case for emphasising unstructured data in financial services
First-movers have begun acknowledging the capability of unstructured knowledge data to produce bottom-line results. However, many financial institutions still treat client experience as a compliance process — taking simple survey scores to register agent delight. Nevertheless, to overcome the challenges of unstructured data in financial services, financial leaders must exhibit a strategy to leverage client data to solve industry challenges.
Leverage the right technology for automated financial reporting
Modern financial institutions and the banking sector should look for solutions that convert speech into text and deliver industry-tuned case models that can identify compositions across all text-based unstructured data sources for better automated financial reporting.
Financial automation solutions exist to examine key drivers of client experience and quantify their effect on functional results, like the first call resolution and running time. Such insights can considerably decrease the managerial effort when streamlining capacity planning or service recovery.
Plan to transform the data opportunity
Technology is a vital enabler that can help financial institutions take the right step towards automation. Data-rich financial institutions usually find that clients recommend fixes that might lower conflict. Each of those proposed measures has the possibility of evolving as a growth initiative.
Nevertheless, financial institutions will not witness change without the capability to take action. Therefore, it becomes essential for financial institutions to move beyond owning mechanisms to facilitate project prioritisation, where even early adopters can look at financial automation. Some fundamental use cases here comprise sentiment data analysis that triggers the most suitable actions in systems like financial document automation and client help tools.
Offer analytics for extracted content
In most circumstances, extraction is not the absolute answer. Instead, financial service organisations these days like to derive practical insights from unstructured data / extracted text. Document AI (Artificial Intelligence) provides characteristics like classification, clustering, context comprehension, summary, learning graph, sentiment research etc., to make the text data gather insights for financial businesses to leverage document automation.
Leverage NLP (Natural Language Processing) in financial automation
NLP, also known as Natural Language Processing, assists financial institutions in locating useful data and actualities from unstructured documents and optimises the examination of complex records such as real estate descriptions, statutory orders, contracts, etc.
Manual research, the typical solution to acquiring better insights from these sources, is incorrect, unreliable, and risks missing crucial attributes.
The capability to explore these and other data sources efficiently and gain insight from unstructured data can guarantee that financial institutions make sound choices regarding existing and future clients.
The Bottom Line
While the data transformations bring challenges for modern financial service organisations, the possibilities are tremendous. Furthermore, unstructured data is also a considerable challenge for large financial organisations.
The challenge with the institution is not just restricted to collecting and gathering data but also acquiring insights from it. Modern AI (Artificial Intelligence) solutions designed by a competent technology partner can readily facilitate financial institutions to bring order to their data while also acquiring valuable insights.
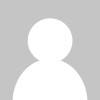
Leave a Reply