We are all adjusting to the newfound necessity of prioritizing an ever-increasing flood of quantitative insights as we live in the era of information. By following the never-ending stream of media, research, blogs, tweets and other social media, it is possible to gain insight into macroeconomic patterns, how marketplaces change over time and even how corporations perceive and adjust to their environment.
Textual data, such as surveys and reviews, as well as interview transcripts and other forms of textual quantitative insights, are used by corporations, government agencies, researchers and educational organisations to gather information. Even though human beings are incapable of reading, gathering and evaluating millions of pages of text, natural language processing (NLP) breakthroughs have made it possible for anyone to spot trends and discern a person’s emotional state from a vast number of words or phrases.
The capacity to sift through a large amount of data and uncover the most important insights for your firm has become a strategic advantage since data is now viewed as a corporate asset. NLP trends can help organisations and investors get a competitive advantage by unearthing insights and patterns hidden in millions of pages.
Let’s take a look at examples of how natural language processing might assist you in quantifying human language and why that’s important in your research.
1. Validate C-Suite Interest on a Trend
How Do C-suite Leaders Use NLP to Learn about the Density of Keywords? Text analytics uses various linguistic, statistical and machine learning techniques to transform unstructured text input into useful information. C-Suite interest might use this information to see how well their marketing campaigns are working or to keep tabs on the most common customer complaints before deciding on how to respond or improve service. Keyword extraction and the detection of structure or trends in unstructured information are two additional ways that natural language processing (NLP) might assist with text analytics.
2. Handle a Large Amount of Data
The availability of large amounts of data is critical for machine learning and even more so for deep learning. On the other hand, quality should not suffer as a result of your decision to prioritise size over everything else. When it comes to data preparation, the most important questions for ML researchers are:
- Do they have adequate data?
- What criteria do they use to assess the quality of their data?
When gathering data on your own or utilising publicly available datasets, these aspects are important to keep in mind. Let’s take a look at each of these concerns one by one.
Defining the size of the dataset
No one can predict how many customer reviews, emails, sentence pairings, questions and answers and other elements you will need to achieve a reliable output. The size of a data set might be challenging to determine, but a few approaches can assist you in this process.
People are constantly working on NLP projects, and their findings are being published in academic journals and blogs. Search for specific solutions that will at the very least provide you with an estimate. Make use of your knowledge or consult with domain experts to accurately determine how much data is required to convey the intricacy of the activity.
Using statistical approaches, it is possible to determine the sample size for any sort of study. Consider the number of features (with x percent more instances than the total number of options), model parameters (with x examples for each parameter), or classes.
These methods are unreliable, but they are still widely used and will get you started.
Evaluation of the quality of text data
In different areas of application, there are differing perspectives on what constitutes high-quality data and what does not. In natural language processing, one quality measure is particularly important: representational.
Representational data quality indicators consider the ease with which a machine can interpret the text. Within the dataset, the following issues have been identified:
- Data that was incorrectly calculated
- Grammatical and punctuation errors
- Similar words spelt differently
- Sentences with a long average length
3. Gaining Different Insights
Understanding consumer feedback and identifying your company’s strengths and flaws are critical to the success of any organisation. Website evaluations, chat interactions, conversation transcripts and social media comments are all examples of information that firms now have access to that can provide them with a search summary.
The use of natural language statements or question fragments made up of many keywords that may be parsed and ascribed a meaning opens up more chances for individuals to examine their data in new and interesting ways. It has been demonstrated that using language to explore data increases both the level of accessibility and the barrier to analytics across businesses, even for those outside the expected group of analysts or software developers.
4. Sentiment Analysis Using NLP
If you wish to assess whether data is favourable, negative, or neutral, sentiment analysis (also known as information extraction) could be a natural processing technique to use. Positive and negative feelings in the text can be detected through sentiment analysis. Customers’ perceptions of a brand can be assessed using this technique in social media posts, as can the company’s reputation.
The sentiment analysis method has three possible applications:
Rule-based Approaches
These systems typically use rules written by humans in order to determine the subjectivity, polarisation or subject matter associated with an opinion. Stemming, tokenisation, part-of-speech tagging, and parsing are all examples of NLP techniques that may be used in these rules.
Whenever there are more positive words in a passage, the algorithm produces a positive sentiment and vice versa. As long as there are no odd or even numbers, the algorithm will return a neutral feeling.
Automatic Approaches
Unlike rule-based systems, automatic approaches do not rely on a set of predetermined rules but rather on machine learning.
Hybrid Approaches
Hybrid systems incorporate the best aspects of rule-based and automated systems. One major advantage of using these approaches is that the findings are frequently more accurate than with traditional methods.
NLP Applications in the Investment Sector
NLP is being used across the financial world, from consumer banking to hedge fund investment, and it is becoming increasingly popular. Natural language processing (NLP) techniques such as sentiment analysis, question-answering (chatbots), document categorisation and topic clustering are employed when dealing with unstructured financial data.
A financial system that can make intelligent decisions in real-time can be designed using NLP and machine learning approaches. A company’s evolving character can be tracked with NLP to assist in creating solutions that enhance cash flows. Investment operations, for example, can benefit from NLP in the following ways:
- Improved detection and response to fraud or money laundering operations.
- An increase in adherence to rules and regulations.
- Operations have become more efficient.
- Improved personalisation of the experience for customers.
Conclusion
In recent years, natural language processing technologies have become significantly more dependable, consistent, accurate and scalable, allowing financial decision-makers to gain a comprehensive understanding of the market and make better-informed decisions. In the financial industry, natural language processing (NLP trend) is being used to drastically reduce tedious activities, speed up deals, identify risks, interpret financial emotions and design portfolios while automating audits and accounting.
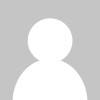
Leave a Reply