Artificial intelligence is becoming one of the megatrends. Businesses are keen to utilize the full potential of AI to improve the efficiency of their service. The healthcare sector naturally accumulates a vast data set in the form of health records, images, clinical trial data, claim data, etc., these data are used to make better business & clinical decisions.
As data stays at the nucleus of business and clinical decision-making in the healthcare sector, AI can help the healthcare industry automate several mundane tasks and also have a deeper insight into data like never before with human resources. In fact, a Business Insider Intelligence report on AI in healthcare states that “spending on AI in healthcare is projected to grow at an annualized 48% between 2017 and 2023.”
In this post, let’s see how AI in healthcare can help in process automation and in healthcare insurance.
Problem with legacy systems & advanced data
Being flooded with a massive amount of data, the healthcare industry struggles to utilize the data and safeguard them. The inability to properly manage the data leads becomes a big challenge for clinicians to provide better patient-centered care and accelerated decisions. Following are some of the biggest challenges the healthcare industry faces due to the old legacy system.
- In healthcare insurance, the biggest challenge with the legacy system is data validation. The National Health Care Anti-Fraud Association (NHCAA) estimates that the financial losses due to healthcare fraud are around 3% and sometimes as high as 10% in certain states, which is approximately valued at more than $300 billion per year.
- Managing and validating all the data manually inherits errors in the data in one form or the other. Using machine learning and AI, healthcare organizations can easily identify patterns and flag unusual claims. A McKinsey report states that German health insurers alone could save about EUR 500 million each year by deploying Artificial Intelligence.
- Ensuring that the data collected are in compliance with the legislative acts and standards is crucial. The penalties for HIIPA violations can stand anyway from $1000 per mild accident and $100,000 for serious breaches. Hence, maintaining accurate data that comply with the legislative acts will help heal insurers to avoid heavy penalties.
- US patients and healthcare providers pay a whopping $496 billion in billing and insurance-related (BIR) costs every year and in that $248 billion is spent annually for the administrative and processing cost — states the Center for American Progress. As you can see nearly 50% of the total income is been spent on operations alone. The operation cost was around 31% in 1999 and the value has increased gradually due to the increase in healthcare costs and competitive lower policy pricing.
- 30% of healthcare claims are declined or ignored. Error in the medical billing and coding may result in the denial of the claims. Another statistic shows that 27% of denials are due to issues with patient registration and eligibility for medical claims. The improper maintenance of records makes claims processing difficult.
- Due to the lack of proper information and correlations, insurance companies were not able to provide personalized offerings and services to their customers.
How AI in Healthcare can Accelerate Clinical Decisions
Robust and flexible AI platforms can be trained to specific use cases and help healthcare professionals and insurers to automate the data management process. For instance, Recosense’s trained data intelligence Docusense platform can extract data not only from digital health records (EHR & EMR) but can also extract information from unstructured data like handwritten notes, images, PDFs, and other file formats as well.
With the power of NLP and NLU, the AI bot can extract error-free information, and update the information automatically to the desired system/software. All the extracted information will be correlated into a meaningful knowledge graph, which can be used to visualize & extract required information within minutes. Since healthcare professionals and insurers can get desired information with simple queries and predict the desired outcome in advance. Which in turn will help the users to make better data-backed decisions.
Now that we have enhanced context of the data, we can have a better understanding of the information. Plus, we can also quickly validate the information. AI has several insurance industry use cases. Following are some of the use cases for health care insurance-
Faster clinical operations
With the help of AI’s advanced search options, the operations team can quickly search and validate the medical coding. Take forward clinical studies and medical record maintenance easily. Healthcare professionals can quickly mine through the notes and provide better service to their patients.
Automated data entry & validation
As mentioned above, efficient platforms like Recosense can extract accurate information from both structured & unstructured data. The extracted data will be stored in an easy-to-access digital format. Users can map the data to the desired fields so that the extracted information will be stored in the desired fields on the system we want with minimal human intervention.
The AI system can validate the extracted pieces of information as well. It can automatically notify about missing fields, and information such as claim number, ID, and signatures. With the automatic validation in place, the records will have a uniform format and make sure no information is left out.
Quick Claims Processing
Claims processing includes processing of vast amounts of data and validating them. Hence, it takes time to process the claims. It is crucial to make the process quick and also accurate. The AI system can process the claims files quickly and indicates the key output to the insurer. Based on the information the agent can perform claims processing quickly.
According to McKinsey, the use of AI process automation in medical insurance ensures a 30% reduction in claims processing costs.
Policy denials & appeals prediction
One of the biggest advantages of deploying AI is identifying patterns. With the predicted patterns, the deep learning system in the insurance claim analysis can anticipate which all claims can be denied.
For instance, if a policy is likely to be denied because of missing medical detail, it will be notified to the agents and they can proactively get in touch with the customer to avoid denials in the future.
Similarly, the AI can identify the appeals that are more likely to be denied from past data. With the anticipated data, the insurers can proactively avoid those appeals at the beginning stage of the process and save time & resources
Fraud detection
With ever-increasing claims, an automated AI system can help healthcare insurers easily identify suspicious claims. The AI bot identifies the pattern from the historical data from the claims audit and flags suspicious claims to the agents. The agents can have a deeper look at the claims manually and decide on the proceeding.
Based on the rule book, the AI bot can be trained so that the bot knows which claims are false, and which need to be flagged.
Risk assessment
Reconsense AI can be used to assess the risk of insuring a particular individual or group of individuals, allowing healthcare insurance companies to better understand the level of risk involved and adjust premiums accordingly.
Recosense AI solution for Helathcare
AI solutions for healthcare can automate many of the time-consuming and manual tasks involved in healthcare insurance, such as claims processing and fraud detection. This can help reduce the workload on employees, allowing them to focus on more complex tasks.
Reconsense AI solutions for healthcare can –
- Helps in true digital transformation. All the unstructured data from documents, PDFs and images (eg.provider contracts, member contracts, prior authorization requests) will be turned into digital structured data.
- Interpret meaningful information from digitized documents with trained AI models.
- Automated data validation and notifies missing data like applicant details, claim number, Patient ID, Billed amount etc.
- Ensures compliance against Insurance Guidelines
- Automated claims audit and use pattern matching to identify deviation measurement, anomalies and discrepancies, insurer specific claim possibility, etc.
- Efficient claims management and suggestions to improve claim success.
- Recommendation of physician medical reports from successful claims
- Auto-suggestion based on similar claims success/rejection patterns.
Impacts of using AI in healthcare
The AI system can automate 60% to 70% of the basic chores. Some leading healthcare organizations have already deployed AI automation in their systems and are seeing improvements in their processing and revenue.
- Care1st Health Plan Arizona reduced the time needed to process a single claim from 20 seconds to just 3 seconds by adopting an AI system
- Baylor Scott & White Health (BSWH), a health system that serves 52 hospitals across the USA, introduced an AI-powered claims evaluation mechanism. With the AI system, they can now make 70% of estimates without human involvement.
- Anthem, one of the largest health insurers in the US, uses AI to identify fraudulent claims and reduce the amount of time it takes to process claims. The company also uses AI to analyze patient data to identify those who may be at risk of developing chronic conditions.
ReconSense AI platform can be a valuable tool for healthcare insurance companies to improve efficiency, reduce costs, and provide better service to their customers. The flexibility of the AI system will help healthcare insurers to scale the process rapidly as per the requirement. Plus, the simpler system makes deployment into any system easier.
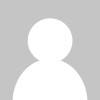
Leave a Reply