Nowadays, as the world goes digital, the popular notion in the finance industry is that with the integration of Artificial Intelligence and machine learning with sentiment analysis, you can forecast future events by analysing the past, leveraging the finance data. They can mimic cognitive decision-making while avoiding the behavioural biases that humans are prone to.
According to research, AI in fintech commanded a market value of $7.91 billion in 2020 and is predicted to reach $26.67 billion by 2026 (a CAGR of 23.17 per cent). Several finance giants across the globe have already put their steps forward to invest in business units focused on AI and ML implementations that could predict their clients’ sentiments to market dynamics. Indeed, AI and ML tools are regarded as the most influential facilitators of competitive and strategic edge in the finance industry. Here is how AI empowers the finance world in sentiment analysis to upgrade their products and services.
Top 3 AI Applications for Financial Sentiment Analysis
These are some of the most prominent AI-backed sentiment analysis applications the finance industry could harness –
1. Exploring relevant data and
information :
Financial institutions have traditionally amassed an enormous volume of data and information records. Some conventional banks even retain the required resources for AI-based sentiment analysis projects. Recognising the power of AI in information search and discovery applications for the finance sector is crucial for proficiently exploring enormous data banks to generate valuable, actionable business insights.
AI-backed software solutions can help financial institutions power a data collection tool that could serve as a search engine for the financial market progressions. The database could then be revised regularly to develop a comprehensive index of all internal documents, such as the latest deal signings, public corporation filings and conference call transcripts, using NLP and machine learning. The search engine then decrypts these documents’ subjects, notions, and theories to index relevant investing information.
In banking operations, the same technologies that fuel the performance improvements in machine learning models of search engines are being exploited. Object recognition systems analogous to those used in search engines, for example, are utilised to detect news and social media interactions pertinent to publicly listed corporations. The motivations for banks to access additional data may expand as more companies employ NLP and machine learning approaches.
2. Generate reports using NLG :
Using natural language generation (NLG), Al-powered software solutions can assist financial firms in conveying insights gathered from structured data. Users can incorporate various tools into their ongoing business operations to help with functions like report generation. For example, a financial firm that employs human analysts to provide narrative reports, like a comprehensive sales report, could automate the compilation of those documents by training the software feeding current reports.
Analysts and technical experts must train the NLG algorithm to obtain reliable and precise reports. Then, the software may learn to recognise correlations in the reports and determine which portions of the reports are the most substantial, allowing firms to ultimately generate reports in natural language with the optimum sentence phrasing, formats and layouts.
Also, banks and financial entities can employ AI capabilities to automate the process of evaluating and gathering insights from customer surveys using NLP. Various software tools use NLP to classify the responses from the customer survey data. They then determine the tones-favourable, critical, or neutral in the content.
3. Automate business processes using NLP and ML :
Text and sentiment analysis systems assist banking and insurance businesses in assessing client and industry sentiments. They are devised using machine learning and NLP approaches. By integrating their text and sentiment analysis tools, APIs allow users to automate internal processes requiring unstructured data analysis and interpretation, such as text documents or photos.
Then the tools employ NLP and image recognition to train models utilising statistical information from banking and financial accounts. The models get trained to categorise, consolidate, and analyse data dynamically.
AI-backed solutions like PreCeive, a plug-and-play software tool, allow financial analysts to gauge customer mentality, reactions, or sentiments through survey questionnaires or other customer engagement data such as call transcripts, emails, or SMS. PreCeive then uses natural language processing (NLP) and machine learning to categorise and uncover insights about individuals, corporations, concerns, products, and activities from text-based datasets. Several businesses can deploy such tools to automate their workflow and offer insight to their customer support and marketing divisions obtained from emails or any other text interaction.
Conclusion
The AI-enabled solution providers accentuate two principal approaches to sentiment analysis in finance. The first one is the development of powerful search engines that can seep through huge volumes of news, microblogs, and social media content with the capability to index and contextualise the most relevant details from the industry point of view. The other involves leveraging existing data repository and corporate documents to comprehend, categorise, and produce concise reports from raw data reflective of favourable, critical, or balanced sentiments.
AI for sentiment analysis cannot be a one-size-fits-all solution to the issues that banks and financial institutions encounter periodically. Instead, industry leaders can leverage it as an instrument that can assist them in exploring ways to enhance and refine insights and findings that already prevail in their data. One thing to keep in mind is that the formulation and establishment of use-cases for tailored AI integrations can take three to five months on average.
The performance, efficiency, and reliability of AI tools are only as perfect as the quality of data and information they consume. Moreover, the models would still need fine-tuning for reliability and accuracy long beyond that timescale.
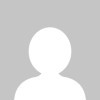
Leave a Reply