In today’s age of digitization, companies are betting big on Natural Language Processing to up their finance game.
NLP is a component of AI that utilizes machine learning algorithms to empower computer systems to comprehend and interpret human language. NLP is most commonly linked with initiatives to improve human-to-machine interactions, such as a customer support chatbot or a virtual assistant.
Today, finance is driven by data. The most crucial data is present in textual form in records, texts, websites, forums, and other places. Finance professionals spend a lot of time reading analyst reports, financial print media, and other sources of information.
Techniques such as NLP and ML could be leveraged to develop the financial infrastructure and thus help make data-driven, informed decisions in real-time.
5 Use Cases of NLP in the Finance Sector
Though NLP has a broad array of applications in the financial services industry, five, in particular, stand out:
1. Unstructured Data Utilization
In the financial services business, dealing with a flood of data is a common occurrence. Research and analytics reports, corporate filings, and transcripts of quarterly revenue are just a few of the financial resources traders, investment managers, financial analysts, and other professionals need to paddle through. Also, the finance data is stored in varying formats like pdf, XML, HTML, web, feeds, etc.
This piling of unstructured data adds to the problem of analysis, making it more time-consuming and tedious. Consequently, imperative finance data capable of providing in-depth insights to build future strategies may remain underutilized and thus influence decision making.
Natural language processing (NLP) allows you to glean valuable information from stuff that is underutilized. You could train NLP models to analyze unstructured data, content, and information to address concerns or trends that could influence financial markets.
NLP aids in the compliance processes of banks across the globe. Unstructured data labeling makes searching reams of digital documents easier, enabling compliance authorities to evaluate whether standards and regulations get implemented.
2. Efficient Text Analytics With NLP
Text analytics is the process of extracting qualitative, insightful structured data from unstructured text, which has grown in importance in the financial industry.
Sentiment analysis is one of the most commonly used objectives of text analytics. It is a technique for determining the underlying sentiment and extract key financial entities by understanding the context from a piece of text.
By utilizing the NLP engine for text analysis, you could remodel unstructured data sources that investors regularly consume into a single, enhanced format optimized particularly for financial applicability. This smart format can be convenient in producing impactful data analytics by enabling readable structured data and effective data visualization, thus increasing the efficiency and accuracy of data-driven decision-making.
Text analytics is primarily used for risk management and alpha generation in the finance world. Institutions like the Bank of America and JP Morgan Chase rely on this technology. Compliance and risk managers, data scientists, quantitative investors, and many others utilize it for scanning through financial documents, thereby gaining imperative insights.
3. Financial Document Analyzer
Using AI technology, users can incorporate their document finance solution into current workflows without interrupting the existing methodologies. NLP empowers finance professionals to read and comprehend large volumes of financial documents automatically.
Businesses can train NLP models utilizing their existing documentation resources. Then, the NLP-backed financial statement analyzer swims through hundreds of these documents to extract and consolidate the most relevant, insightful information.
Furthermore, NLP is instrumental in creating a search engine for financial market developments. There are tons of documents stored in the databases of financial institutions. The NLP-empowered search engine retrieves the elements, concepts, and notions present in these documents to obtain valuable investment data. The system then displays a summary of the most relevant information for search requests from financial firm employees on the search engine interface.
Banks that utilize this technology include Deutsche Bank, Axis Bank, Federal Bank, etc. Some fintech companies use it as well, such as Capital Float, Flipkart, Edelweiss, etc. to automate processing huge volumes of documents and extracting key concepts and data fields from it.
4. Content Enrichment
Financial firms could harness content enrichment to compose better investment management and improve risk management and compliance. Content intelligence systems are sound in completing and enriching enterprise-wide data with extensive contextual information to entice real, actionable insights.
Content intelligence is an emerging technology that boosts content strategies by delivering data-driven analytics on content and its influence on consumers. It entails both direct and indirect financial gains from content marketing initiatives, such as decreased squandering and increased ROI.
These tools leverage the NLP technology to:
- Assess the tone of your content, spot patterns, and make data-driven decisions.
- Figure out what the content’s goal is and how to route it to the apt topic.
- Generate and curate content for your engagement platforms.
NLP helps achieve content enrichment objectives in the finance sector by:
- Identification and differentiation of the most appealing thought leadership blogs from the competition.
- Delivering personalized customer experience through targeted client-specific content.
McKinsey, a management consulting firm, has a content enrichment and intelligence model that verifies data content and performs quality and consistency checks. This allows them to help their clients with insurance, wealth, and asset management.
5. Reporting and Omnichannel Customer Engagement
The finance industry has lots of data to sink in, making it challenging to monitor transactions. Natural language processing serves the purpose of allowing financial analysts to obtain relevant information through information filtering.
Also, in the financial services industry, client communication is imperative this sector, and NLP tools provide banks with critical information when they interact with customers.
NLP algorithms forecast and detect customer pain points, allowing banks to design policies and services to address these issues. Other financial institutions could take decisions backed by NLP, which speeds up the overall financial reporting process even further.
What’s more, financial firms must provide high-quality services to their customers that necessitate going above and beyond to comprehend customer data and information. NLP analyses customer data, such as social interactions and cultures, to personalize services.
NLP, for example, sifts through social media data and finds conversations that might help them improve their services. Major retail banks like HDFC Bank and ICICI Bank deploy powerful customer engagement tools like chatbots to understand client intention.
Top 5 Key Benefits of Utilizing NLP in Finance
Consider the following benefits of utilizing NLP to the fullest, especially in the finance sector:
1. Efficiency
It is capable of automating large volumes of unstructured content into meaningful insights in real-time.
2. Consistency
A single NLP model can deliver way more consistently than a team of human analysts, each of whom may decipher aspects of text slightly differently.
3. Accuracy
Human analysts might overlook or misread content in voluminous unstructured documents. It gets eliminated to a greater extent in the case of NLP-backed systems.
4. Scaling
Text analysis at scale is possible using NLP technology on a variety of documents, internal processes, emails, social media data, and more. You can process massive volumes of data in seconds or minutes, whereas manual analysis might take days.
5. Process Automation
NLP empowers you to automate the entire process of scanning and extracting actionable insights from the financial data under study.
The Bottom Line
In recent years, natural language processing algorithms have grown considerably more reliable, consistent, accurate, and scalable, providing financial decision-makers with a thorough grasp of the market. NLP is being used in the finance industry to significantly reduce mundane tasks, speed up deals, analyze risks, comprehend financial sentiment, and build portfolios while automating audits and accounting.
Thus, there is no hesitation in saying that natural language processing (NLP) and machine learning (ML) have become the tools of choice for financial analysts, traders, and portfolio managers.
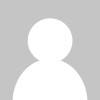
Leave a Reply