The influx of data has long challenged the insurance industry—a cascade of claims, market analyses, regulatory documents, and customer interactions. Amidst this data accumulation lies the need for efficient processing, accurate risk evaluation, and compliance adherence.
Fortunately, the insurance industry has witnessed a remarkable transformation in recent years due to technological advancements. One of the most significant breakthroughs is the integration of Natural Language Processing (NLP), a branch of artificial intelligence, which holds the potential not just to subdue these challenges but reshape the very foundations of the industry.
This blog addresses the challenges faced by the industry and explores how NLP methods are being harnessed to streamline processes, enhance risk assessment, and optimize market analysis.
The Need for NLP in Insurance
Insurers struggle with the complexities of unstructured data, voluminous documents, and the critical need for accurate risk assessment in an industry fueled by data and information. Traditional data handling and analysis methods often fall short due to their time-consuming nature and susceptibility to errors.
NLP emerges as a solution to these challenges, offering a way to sift through massive data sets, extract valuable insights, and drive data-driven decision-making.
- Huge Volume of Data: The insurance sector generates massive amounts of data daily, encompassing customer information, claims records, policy documents, and market trends. There is a massive need to process this data and identify patterns, relationships, and anomalies that may otherwise remain hidden.
- Unstructured Data: A substantial portion of insurance data is unstructured, residing in forms, emails, customer feedback, and other textual formats. The insurers are required to understand customer sentiments, detect fraudulent claims, and personalize services to deliver customer satisfaction.
- Risk Assessment: Accurate risk assessment is at the heart of insurance operations. Vast amounts of data—historical data, customer profiles, and external factors—need to be analyzed to
– Enhance underwriting precision
– Uncover subtle correlations
– Predict potential risks
- Market Analysis: Insurance companies must constantly monitor market trends, competitors, and regulatory changes. They must also extract relevant information from news articles, research papers, and social media, facilitating proactive decision-making and competitive positioning.
This is where the demand for NLP in the insurance sector arises.
Streamlining insurance with NLP methods
NLP houses a variety of methods that empower insurers to unlock insights from textual data. These include two pivotal techniques:
1. Syntax analysis
This structural backbone of NLP is concerned with understanding the grammatical arrangement of words. It encompasses the following techniques:
- Parsing: This technique tags words based on grammatical roles (nouns, verbs). It aids insurers in extracting essential information and relationships from policy documents, claims descriptions, and legal texts.
- Lemmatization and Stemming: These techniques reduce words to their root forms, standardizing vocabulary and grouping related terms. For instance, “running,” “ran,” and “runs” are reduced to “run.”
- Sentence Breaking and Word Segmentation: Identifies accurate sentence boundaries and segments words, even in languages without spaces.
- Terminology Extraction: Syntactic techniques enable the recognition of domain-specific vocabulary and extraction of key terms for efficient indexing, monitoring, document retrieval, and analysis
2. Semantic analysis
It delves into the meanings and interpretations of words and sentences. The various techniques that fall under this are:
- Natural Language Generation (NLG): Converts data into human-readable language, generating coherent reports, summaries, and explanations
- Natural Language Understanding (NLU): Enables computers to comprehend human language, respond intelligently to user input, and drive efficient interactions.
- Named Entity Recognition (NER): Identifies entities like names and dates within the text, aiding in extracting policy numbers, customer names, and relevant dates from insurance documents.
- Sentiment Analysis: Gauges emotional tone, helpful in understanding customer sentiments and detecting issues like fraud.
- Topic Recognition: Recognizes central themes, enhancing data organization and retrieval.
Uncovering NLP use cases in Insurance
Claim Processing:
NLP automates the extraction of relevant information from claim documents, facilitating quicker and more accurate claims processing. It is done through NLP-powered Chatbots and optical character recognition (OCR) models which increase operational efficiency and customer satisfaction.
Report Analysis:
Voluminous market research reports hide valuable insights. NLP efficiently extracts and synthesizes critical data, empowering insurers to react swiftly to market shifts. It ultimately enhances the competitive edge.
Underwriting Automation:
NLP gathers data by extracting relevant information from a multitude of sources—policy forms, customer histories, and external sources—assisting in precise pricing and policy decisions. This automation not only accelerates the underwriting process but also ensures accuracy.
Most importantly… NLP aids in compliance and risk management.
Risk Analysis:
NLP analyzes historical data, market trends, and sentiment in news articles and social media to provide insurers with a comprehensive risk assessment framework. This enables them to make data-driven decisions, optimize premium pricing, and mitigate potential losses.
Compliance Analysis:
Regulatory documents are often dense and labyrinthine. NLP aids in dissecting and understanding these documents, ensuring insurers remain compliant with legal stipulations.
The remarkable benefits of NLP in insurance
The infusion of NLP into the insurance industry yields a spectrum of benefits:
- Data extraction mastery: NLP extracts crucial data from vast text sources and unstructured data, such as claim descriptions, customer feedback, and social media interactions. It replaces manual data entry and reduces errors, improving data quality and a solid foundation for data-driven decision-making.
- Swift document retrieval: NLP’s contextual understanding enhances document search speed. Unlike traditional keyword-based searches, NLP understands the meaning and context of queries, delivering more relevant results. This minimiz
es the time spent on searches, enhancing overall productivity. - Precise risk assessments: By analyzing market sentiments and historical data, NLP enhances the accuracy of risk assessments. It equips insurers with a more comprehensive understanding of potential risks driving more intelligent decision-making.
- Trimmed time lags: Automated NLP processes reduce the turnaround time for claims processing, underwriting, and compliance analysis. This translates to faster claims settlements, quicker policy approvals, and streamlined regulatory compliance. The outcome? Enhanced customer satisfaction.
To summarize
The insurance sector is at the threshold of transformation, powered by the capabilities of NLP. As this technology becomes more sophisticated, its role in redefining the industry intensifies. Natural Language Processing isn’t just about making sense of words; it’s about leveraging the very essence of human communication to drive data insights, streamline processes, and enhance customer experiences. The integration of NLP in the insurance industry predicts a future where innovation thrives, risks are more accurately assessed, and the prowess of technology fortifies the promise of financial security.
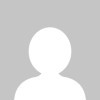
Leave a Reply