Public-company administrators in this modern business world discuss business performance and anticipations with their equity analysts on earnings calls. With the aid of technology, these earnings calls quickly get converted into transcripts that the analysts can use for their future reference or equity research.
The transcripts usually deliver crucial data on market dynamics, a corporation’s performance and a peek into business administrators’ opinions. Nevertheless, due to the unstructured nature of these equity research transcripts, it might become a challenging task for an equity research analyst to perform research or analysis in a scalable manner.
Moreover, it can become quite taxing for portfolio managers to listen to every conference call to perform equity analysis. This is where natural language processing (NLP) can help overcome these challenges. A fully automated NLP algorithm can extract text from transcript statements and execute the obligatory research in just a few seconds that can help boost your number of earnings call transcripts analysed.
What Insights do Researchers use to make Investment Decisions?
NLP can determine trends that keep the investment market driving. News sites, forums, online reviews and social media pages are all transcendent sources from where researchers can look at the trading insights to make effective trading decisions.
Apart from this, NLP can assist researchers in predicting rising trends and preparing for the effect of these differences not just on the leading players within the investment industry but also on all businesses across their industry’s supply chain model.
Some of the trading insights that researchers use to make investment decisions are as follows:
Relationships between entities
In the investment domain, using tags to emphasise the primary topics covered by topic modelling or text is beneficial when researching earnings calls to specify the theme or to compare against similar earnings calls to determine industry trends.
Sentiment analysis
Sentiment analysis is another trading insight that researchers use to make better investment decisions. This insight helps researchers extract the subjective connotation from text adequately to specify its presumption. It is an ideal mechanism for examining unstructured content about a particular business and looking for anomalies.
Scope of AI in the Equity Research Process
With numerous data sources and rising AI applications predicting a paradigm shift in the investment industry, equity research analysts and portfolio managers who previously used to manually filter through enormous volumes of unstructured data for creating equity research reports can now leverage the benefits of artificially intelligent tools. These popular tools, like NLP data processing, help simplify their job.
In addition, gaining insights from filing statements, press releases, financial reports, pitches and presentations, CSR revelations, etc., can be a challenging and time-consuming endeavour. Nevertheless, with AI-based data extraction and automation tools, you can reduce this time and quickly perform large scale data processing.
This use of Artificial Intelligence in equity research can help companies gain relevant insights from current data within a few minutes, and it costs less than the manual processing method. Nevertheless, the real value it provides is by augmenting manual intelligence with effective insights, enabling analysts to channel their actions towards high-value client engagements.
Applications of NLP in Equity Research
Here are some of the top applications of NLP in equity research.
Examining ESG Reports
In modern times, numerous public and private organisations get ranked on their environmental, social and governance (ESG) performance. Businesses usually intercommunicate their fundamental ESG initiatives annually on their web platforms as PDF records.
Stakeholders examine ESG statements to evaluate a corporation’s ESG behaviour. Investment determinations and brand perception can rely on these rankings, and hence businesses must use due care to process data carefully.
NLP can assist in consolidating information into well-defined pieces of data, which can then remain plugged into analytical standards, including market risk estimates and other data domains.
10-K Analysis with the Help of AI
More elaborate than a corporation’s annual report, the 10-K is a powerhouse of data. Thus, the correct estimation of 10-K information would lead to a more in-depth understanding of the business.
As front-office equity research analysts filter numerous 10-K statements and other records in a day, inconsistencies in research might creep in. Using Artificial Intelligence to examine textual data in the revelations made within 10-Ks can broadly cut through this protracted process.
In addition, employing Artificial Intelligence to explore the textual data in the revelations made within 10-Ks can significantly shorten this lengthy cycle. Data extraction instruments can pass through these fragments of texts to gain applicable insights.
Also, a mechanism custom-built for your business and qualified in the scope of your industry can deliver this data to your enterprise applications quickly. A higher number of documents and records can get processed in a limited time frame, and with these new insights, equity analysts can take their time to look at the company’s untapped potential.
The Bottom Line
In a nutshell, NLP technologies present an excellent possibility for multinational investors to acquire relevant discernment that can promote ideal decision-making for a robust bottom line. Easily identifiable, quantitative, structured information has been employed for years to make rational investment determinations, but a lot of other information has stayed primarily untouched.
In addition, private equity research information, earnings calls and 10-K forms are replete with qualitative information and data. Also, correlated with rising information sources such as ESG documents, investment analysis is more problematic today.
After completing research, analysts are left with only a small portion of their time for real research and decision-making. However, NLP data aids in equity research, ultimately strengthening a company’s bottom line.
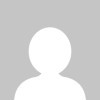
Leave a Reply