The worldwide eCommerce market has expanded by great strides in recent years. However, as the retail and eCommerce landscapes evolve, so does the risk matrix. Rising sales volumes offer a breeding ground for fraud, malpractices and breaches.
To combat such new arising risks and recoup lost profitability and margins, retailers must strengthen security with AI-driven fraud detection systems designed specifically for the retail segment.
What Is Retail Fraud? Why Should You Bother About It?
As in-store and online shopping practices converge, fraudsters exploit the resulting loopholes, devising new methods of assaulting security at every level of merchants’ interactions with buyers. Online stores are particularly vulnerable, with an increasing volume of transactions, orders, and deliveries due to customers’ gradual shift from in-store sales to online purchases.
Inventory shrinkage, bogus cash returns and credit card theft are the most common threats to traditional retailers. According to recent industry research, most losses in the eCommerce space are the consequence of fraudulent card transactions and account takeovers. Nonetheless, the range of retail risks is consistently increasing, and fraud patterns are becoming more complex.
Top 3 Major Types of eCommerce and Retail Fraud
These are some most common types of retail frauds faced by merchants and businesses operating in the domain
1. Inventory shrinkage
Inventory stock disparities in stores can occur due to various factors, including stealing, ticket switching, and clerical errors. Sadly, the staff and supplier activities are a primary cause of such issues. While manual stock-taking provides ample scope for pilfering, low-cost deep learning analytics tools make it relatively simple to detect and avoid stock shortages in real-time, as well as reducing POS inventory loss.
2. Affiliate fraud
Affiliate marketing strategies are deeply associated with this type of fraud. These schemes function by paying a fee to a website blogger or an influencer for promoting a brand and driving followers to visit the business’s website. Affiliate fraudsters use the system to fraudulently receive commissions by using click farms to generate phoney traffic and imitate customer interest
3. Chargebacks
A chargeback occurs when a customer attempts to get the money returned from a valid purchase transaction when they should have claimed a refund instead. Although many chargebacks are deemed “friendly fraud” and are not a malicious effort to extract money, the consequences for businesses are as damaging regardless of the buyer’s intentions. Every chargeback reduces the seller’s profit margin and may result in lost merchandise and high processing fees.
Two Powerful AI Use Cases of Retail Fraud Prevention
1. Predictive Analytics
It leverages analytics tools and platforms for large-scale customers and transactional data to detect fraudulent activity linked to previous incidents of fraud. AI fraud detection solutions backed by predictive analytics can synchronise with retail payment processing infrastructure at the point of sale. Then, ML algorithms that fuel fraud detection systems learn to identify trends and characteristics linked with fraud.
Some examples of fraudulent activities that could be monitored and predicted include transactional analysis and actions such as buying, accounts payable, sales projections, POS, warehouse inventory movement, product returns, etc., and other data across the entity. It can assist you in identifying fraudulent transactions and designing appropriate objectives for case management and interrogation.
2. Anomaly Detection
AI fraud detection systems for retail transactions function by analysing massive amounts of previous and contemporary transaction data to discover underlying motives and detect anomalies. They reveal hidden data trends and patterns that are difficult to decipher without computational power. When an anomaly is spotted, AI-driven anomaly analytics solutions can restrict a user, a transaction, or inform retailers, depending on the documented principles.
Moreover, deep-learning anomaly detection engines can self-learn and consistently optimize algorithms based on previous transactions.
Anomaly detection systems that are highly adaptive to ML and AI can serve almost any retail fraud detection use case. Due to their ability to uncover non-standard patterns owing to the extensive analysis of big data in multiple contexts, they assist businesses in detecting and preventing fraud in physical and online stores.
- These engines analyse multiple ID or credit card parameters using AI and ML algorithms to curb fake ID and credit card transactions.
- The system prevents promo code and loyalty program abuse by catching users who utilize multiple accounts or proxy servers to make unlawful purchases and derive benefits.
- They provide a real-time transaction risk and vulnerability score, enabling them to detect and thwart fraudulent efforts in a fraction of a second during a mobile eCommerce session. ML algorithms examine and interpret the subtleties of specific behavior, screening every user and transaction and sending alerts when anomalies are identified.
Popular brands that implement this use case include Unilever, Stop & Shop and The Home Depot.
Top Benefits of Deploying AI for Fraud Detection and Prevention in Retail
Some key benefits include
1. Quick and accurate detection of potential frauds
AI-backed systems evaluate fraud risks against the customer’s value without compromising with the business policies. They also deliver an analysis that assists brands in identifying anomalies and trends much faster and to precision.
2. Reduces the cost incurred due to fraudulent activities
Retail stores get more time to concentrate on critical business objectives when they don’t have to undertake loads of manual transaction audits and reviews. Without the burden of handling manual reviews and charge backs, fraud analysts, for example, can provide recommendations on how to manage risks connected with new products and extended offers.
3. Impactful real-time data processing
Conventionally, retail fraud detection systems operate in response to past events. It implies that they can only avert the types of fraud that have already occurred. However, AI systems can assess anomalies in real-time and intervene on a fraudulent activity even before the attack.
Conclusion
According to a study, the global fraud detection and prevention market size is projected to grow from USD 20.9 billion in 2020 to USD 38.2 billion by 2025. Artificial intelligence has become an imperative tool in the fight against retail fraud.
Many challenges faced by modern retail and eCommerce businesses get resolved quickly by AI-empowered anomaly detection and predictive analytics solutions. AI technology replaces inefficient, rule-based manual inspections with powerful fraud detection algorithms that discover anomalies in a split second.
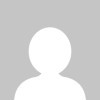
Leave a Reply