Welcome to a world where cutting-edge technology meets manufacturing prowess! In today’s data-driven era, the manufacturing industry is on a quest for innovative solutions that can drive operational efficiency, boost productivity, and elevate product quality to unprecedented heights. Among the … Read More
Author Archives: Recosense
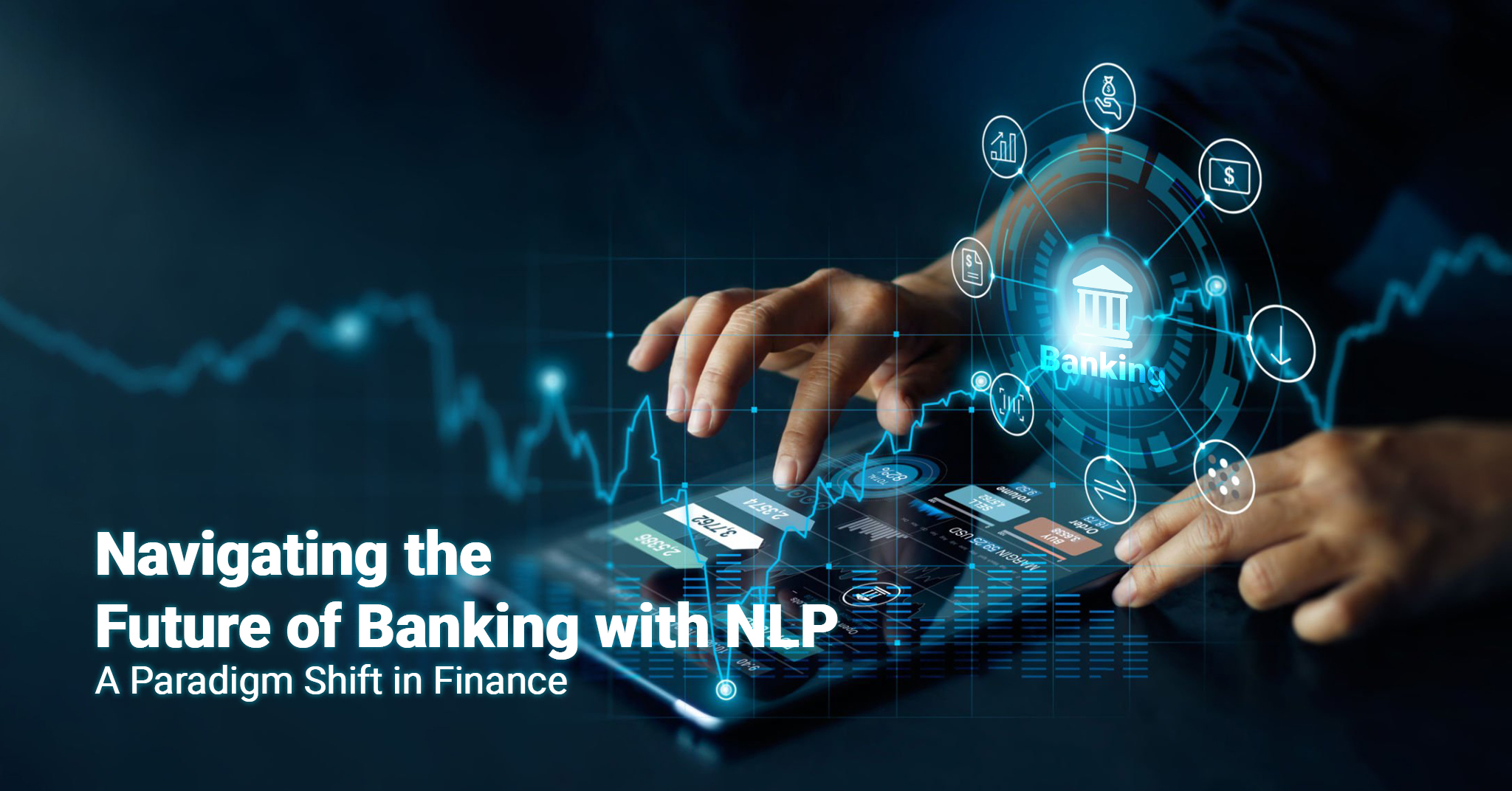
An Analysis of NLP Capabilities in Banking
The banking world is undergoing a remarkable transformation, driven by advancements in technology that are reshaping the industry’s landscape. Among the transformative technologies, Natural Language Processing (NLP) has emerged as a game-changer, empowering banks to harness the power of language … Read More
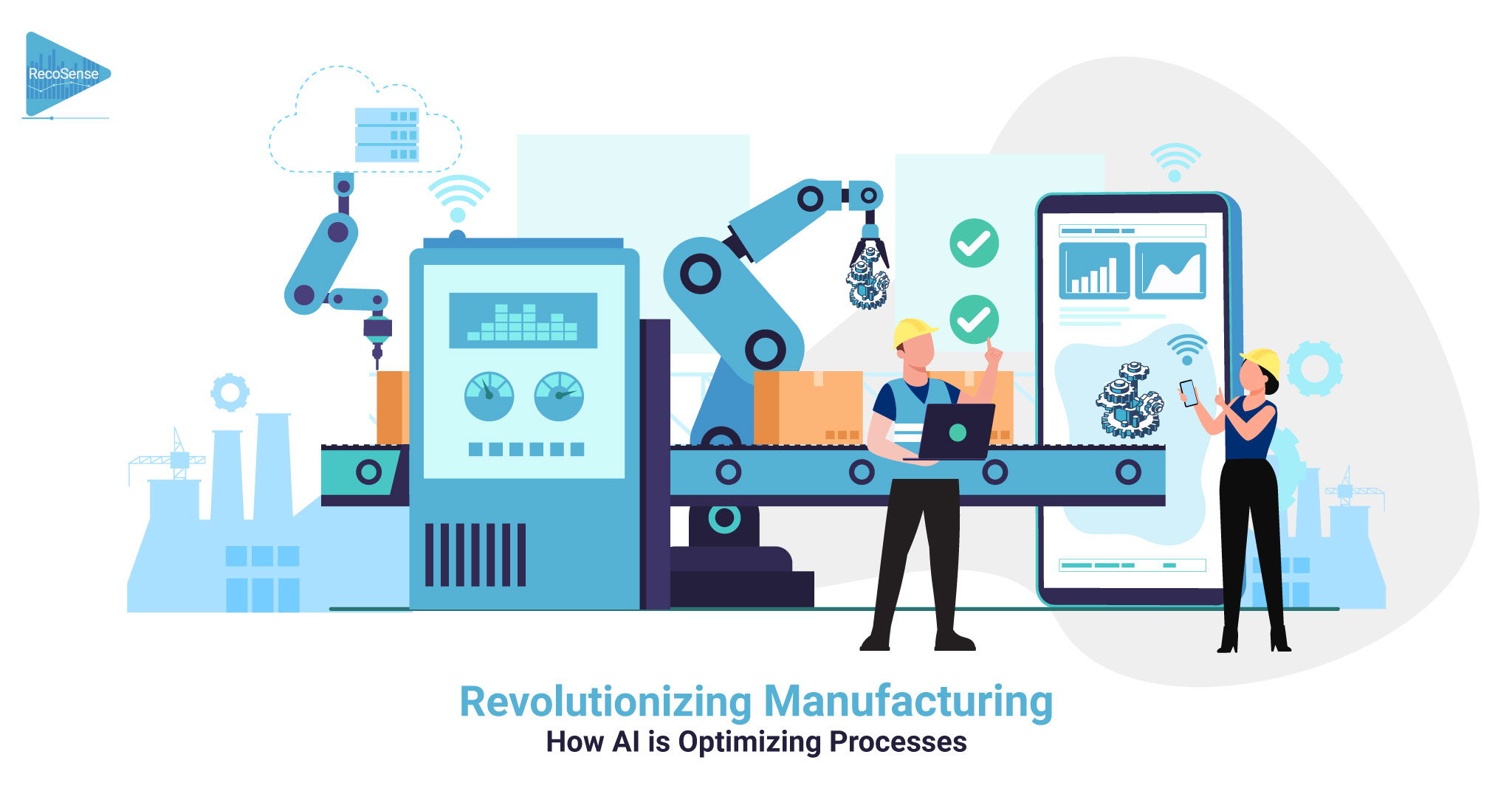
Revolutionizing Manufacturing: How AI is Optimizing Processes
The manufacturing industry is at a crossroads. Digitalization is pivotal in this 4th industrial revolution era, known as Industry 4.0. It connects humans, resources, and information through modern digital systems. With the rise of digital transformation, companies seek ways to … Read More
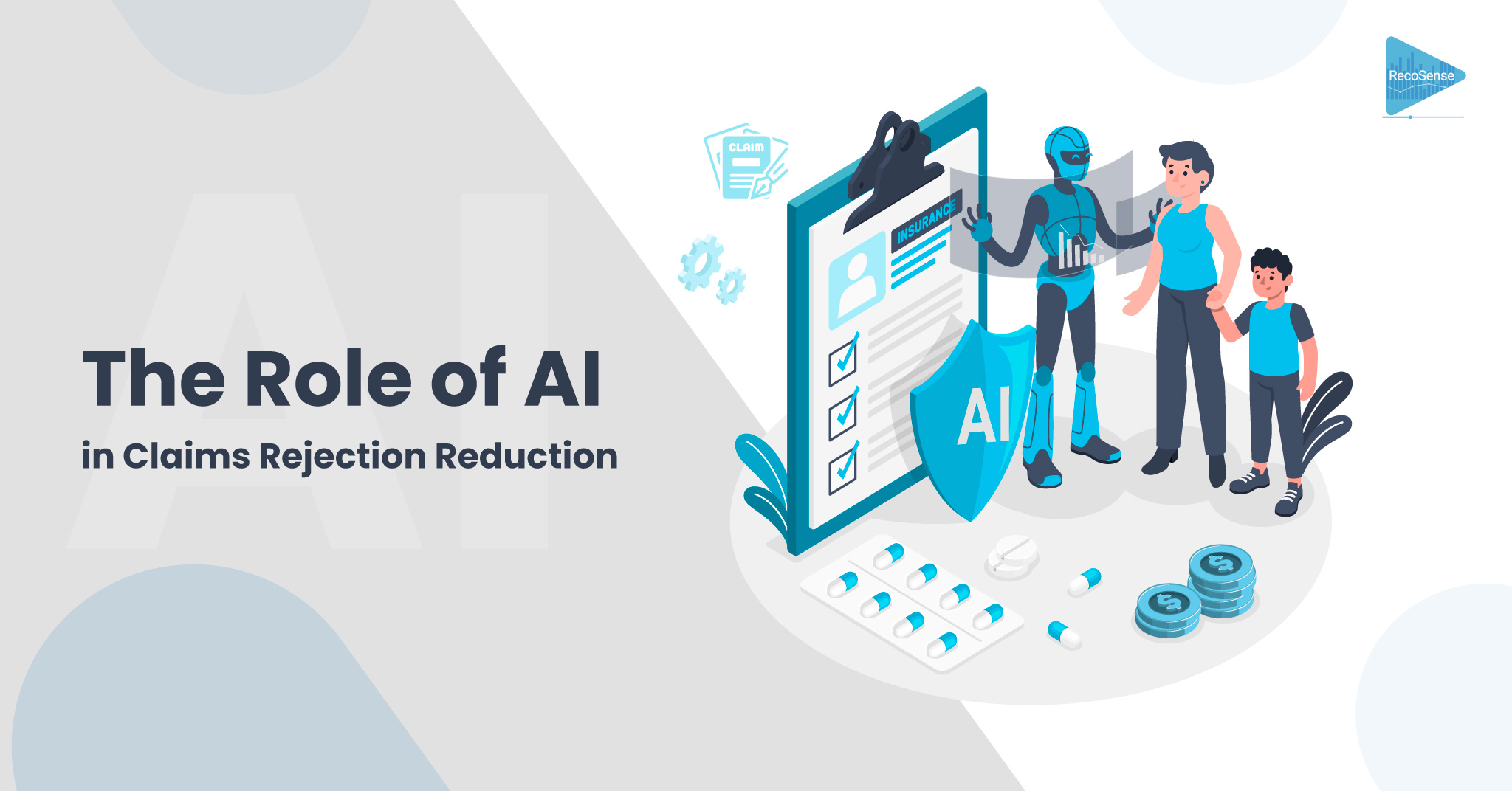
Revolutionizing Healthcare Revenue: The Role of AI in Claims Rejection Reduction
Artificial intelligence is becoming one of the megatrends. Businesses are keen to utilize the full potential of AI to improve the efficiency of their service. The healthcare sector naturally accumulates a vast data set in the form of health records, … Read More
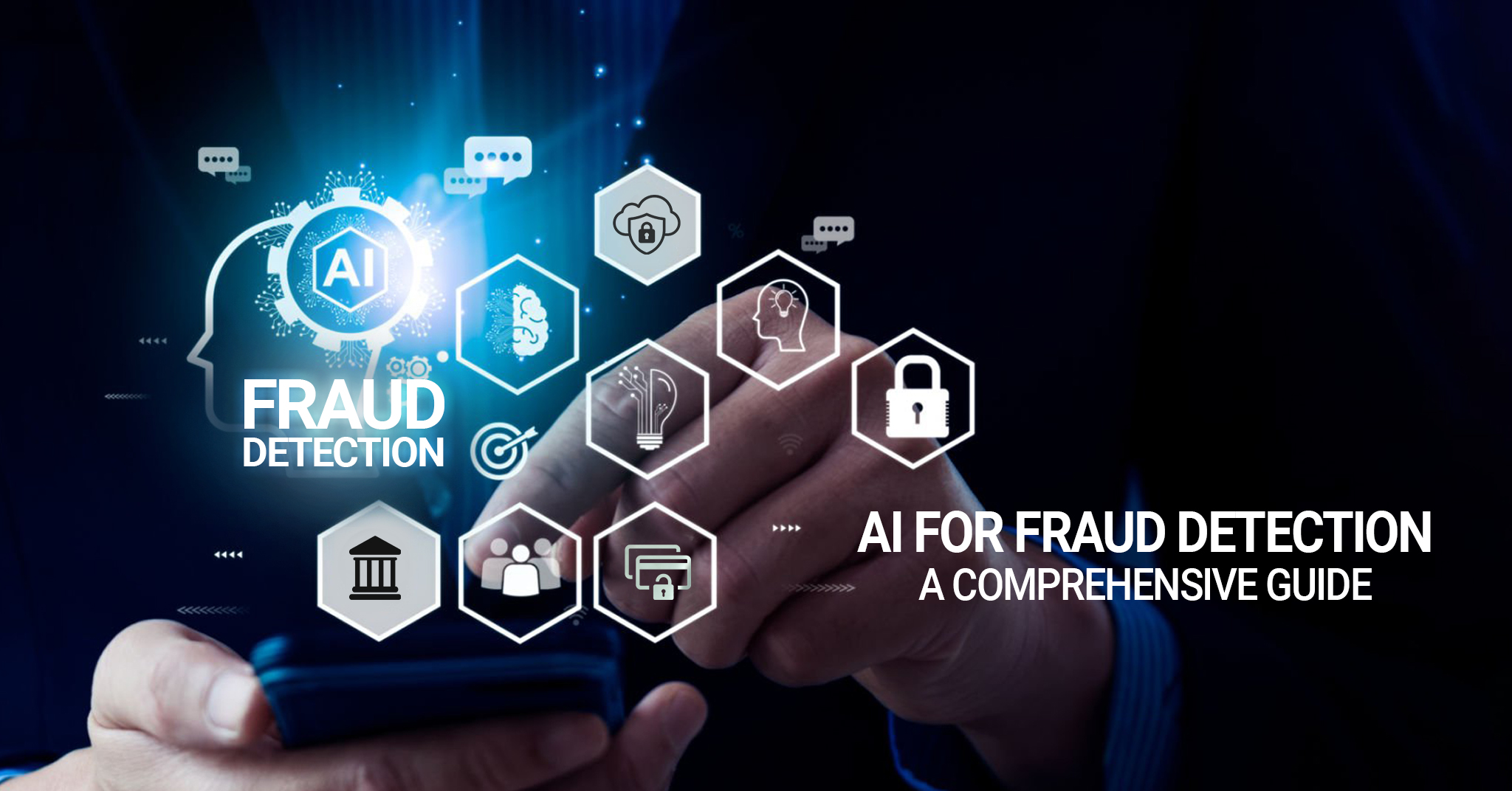
Leveraging AI for Advanced Lending Fraud Detection
Fraud has plagued the lending industry for many years. Fraudulent behavior in lending can take many forms, including identity theft, loan stacking, and money laundering. To combat these issues, lenders increasingly turn to artificial intelligence (AI) for fraud detection. … Read More
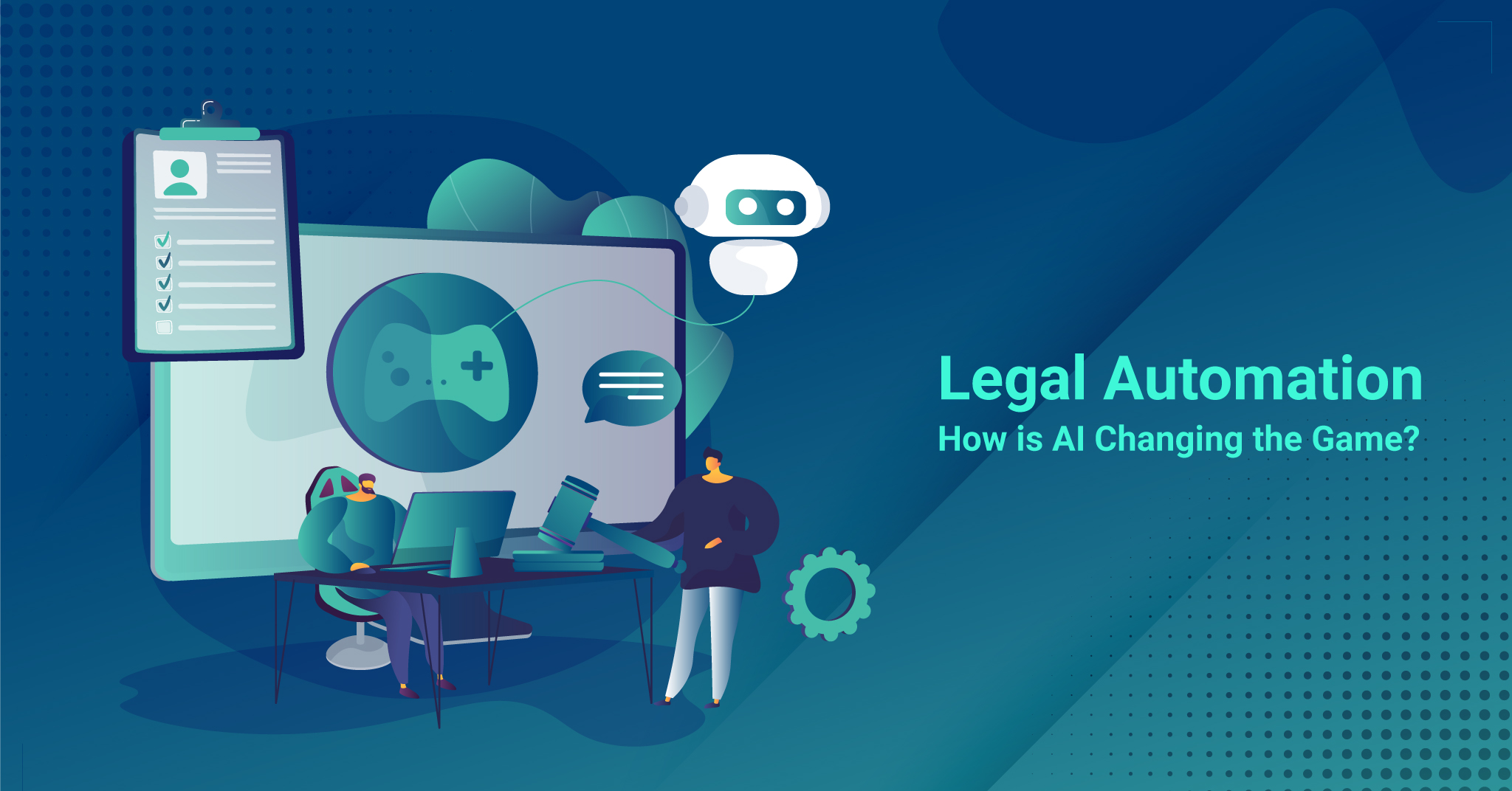
Use Cases of Artificial Intelligence in Legal Industry
The legal field has long been an industry ripe for innovation and the incorporation of new technologies. In recent years, artificial intelligence (AI) and machine learning (ML) have made significant inroads in the sector, and the use cases for these … Read More
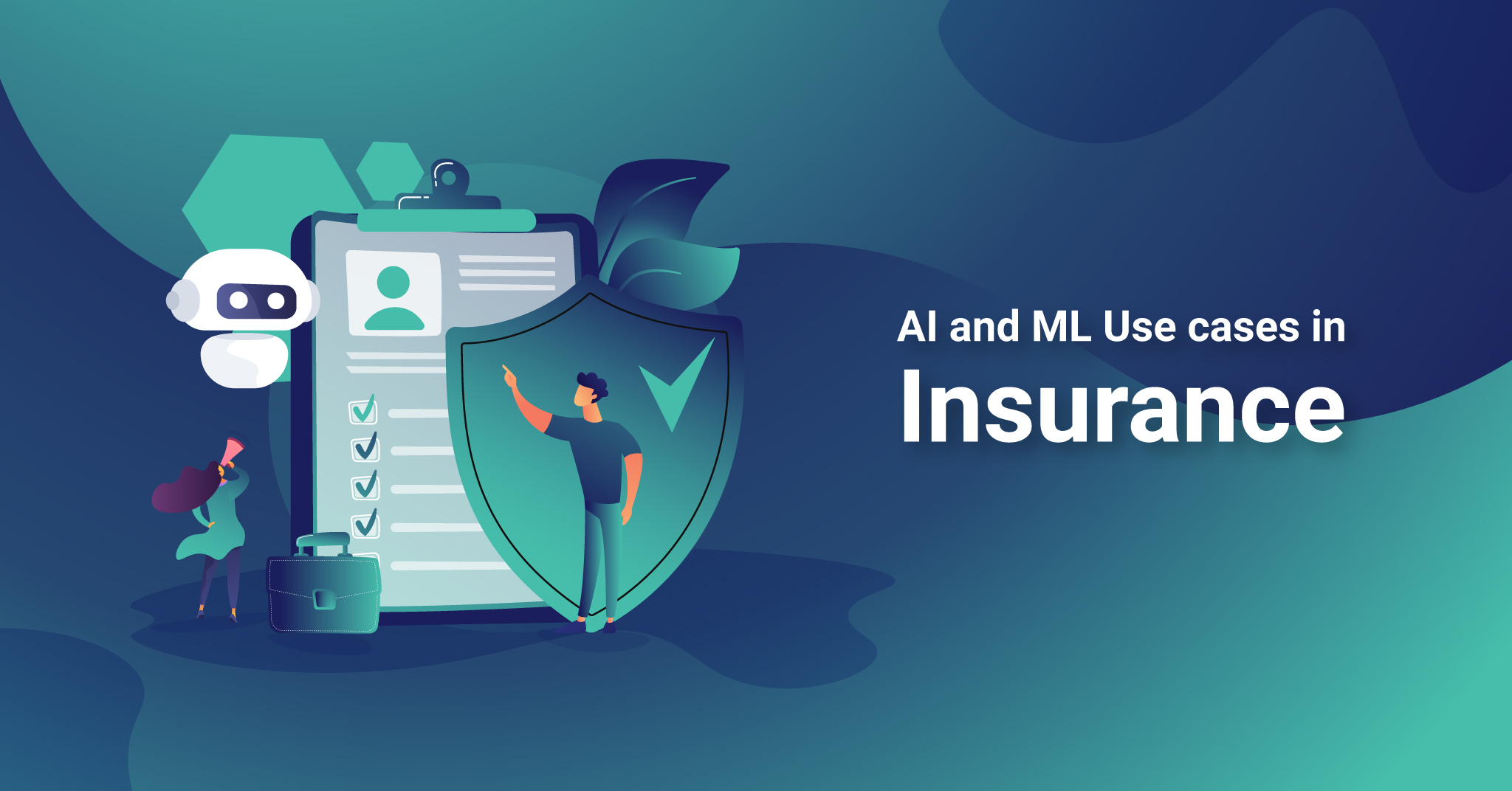
AI and ML Use Cases in Insurance Industry
Changing customer expectations, deteriorating profit margins because of traditional methods, fierce competition, and an ever-changing industry landscape push insurance companies to become innovative. Understanding the demands, the industry players have realized that technology-enabled service is the future. AI and … Read More
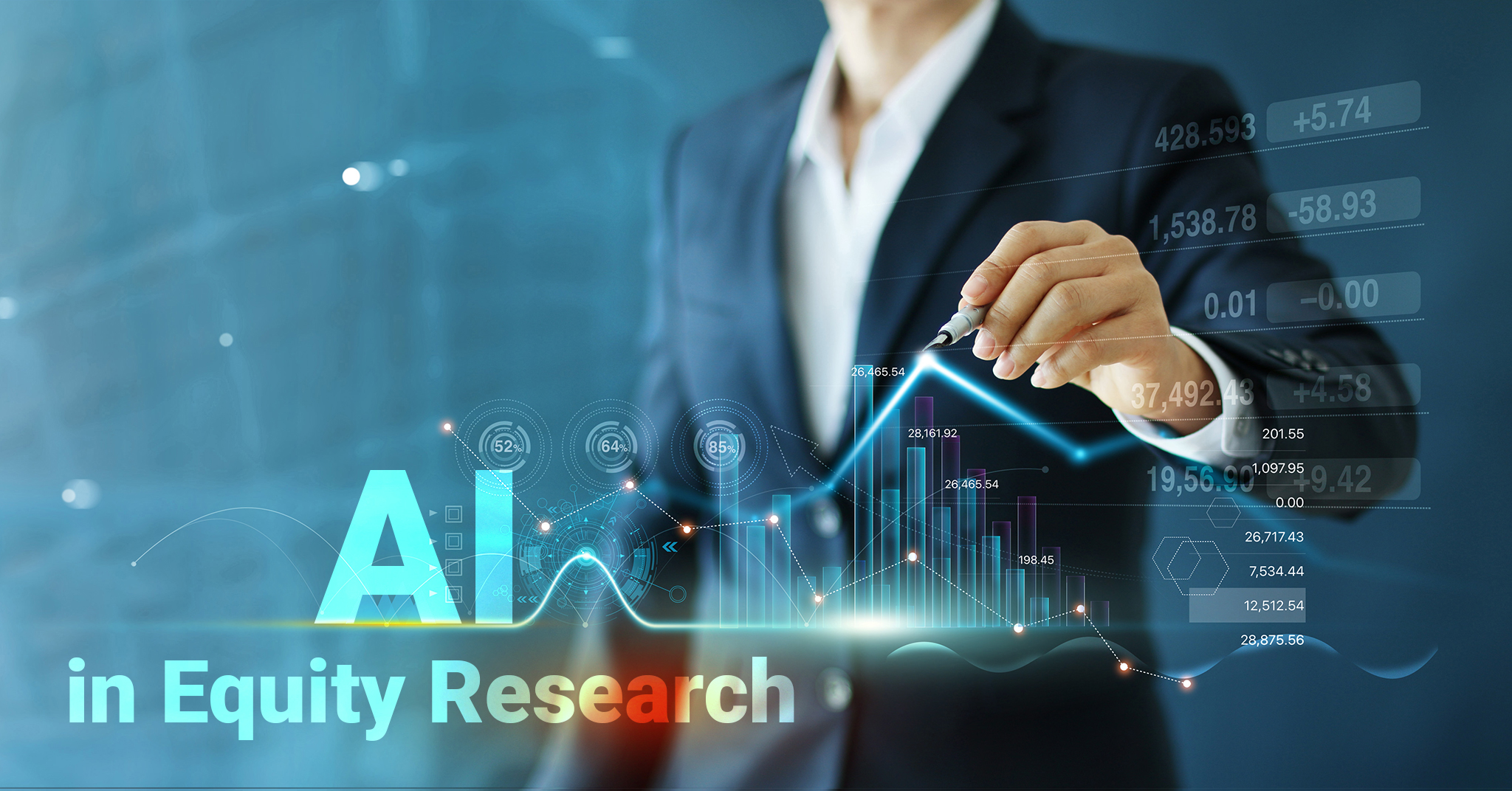
AI in Equity Research
An Overview of AI in Equity Research Private equity firms solely depend on data and how the data is being utilized. The more the equity research team analyzes a company’s data, the better the private equity firms can precisely predict … Read More
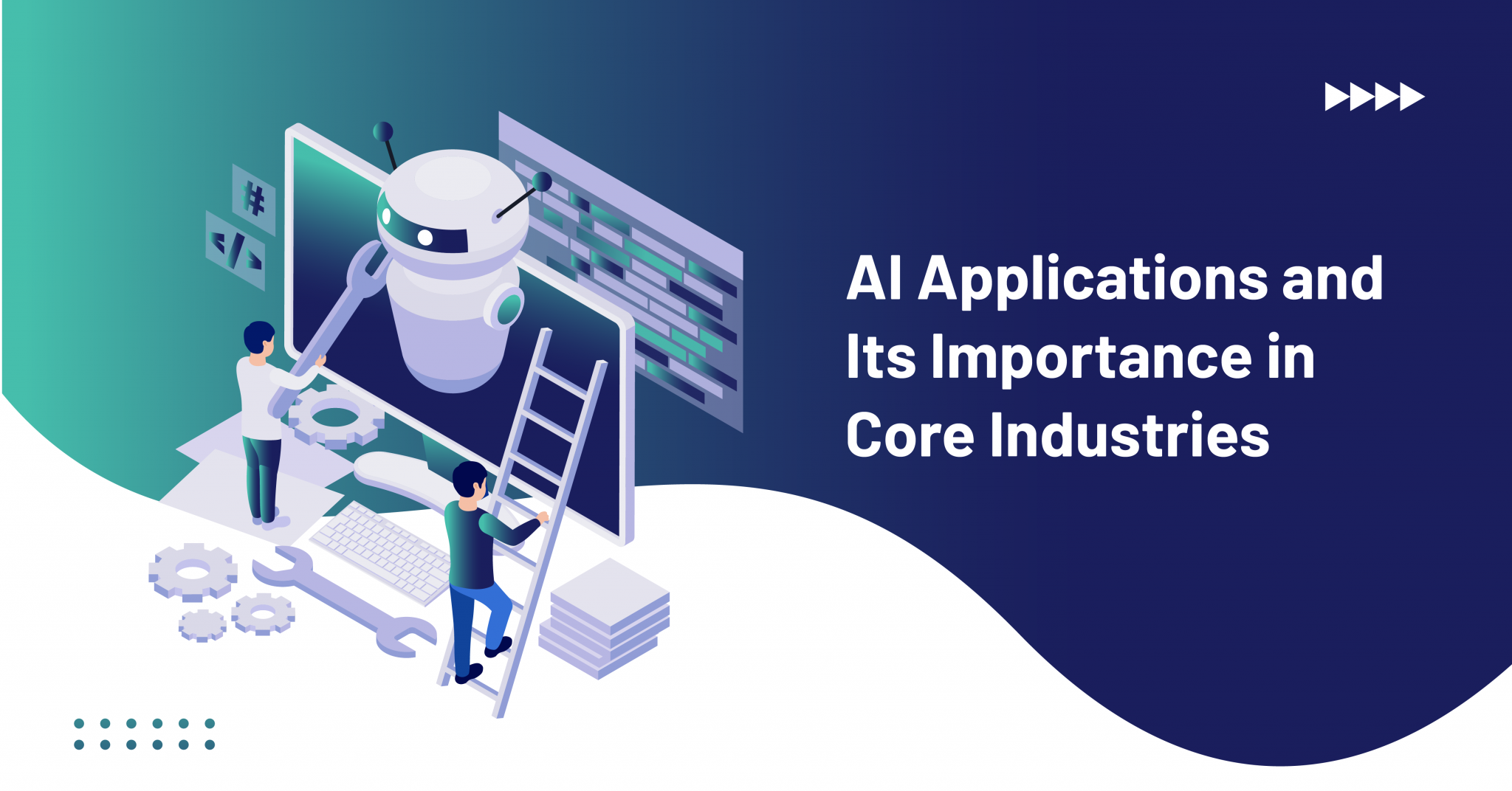
AI Applications and Its Importance in Core Industries
Artificial Intelligence (AI) helps machines think, plan, draw conclusions, comprehend human requests, connect data points and promise real human-machine interaction. Industries and market leaders use AI to drive their businesses and achieve their goals. Today, you can combine different AI … Read More
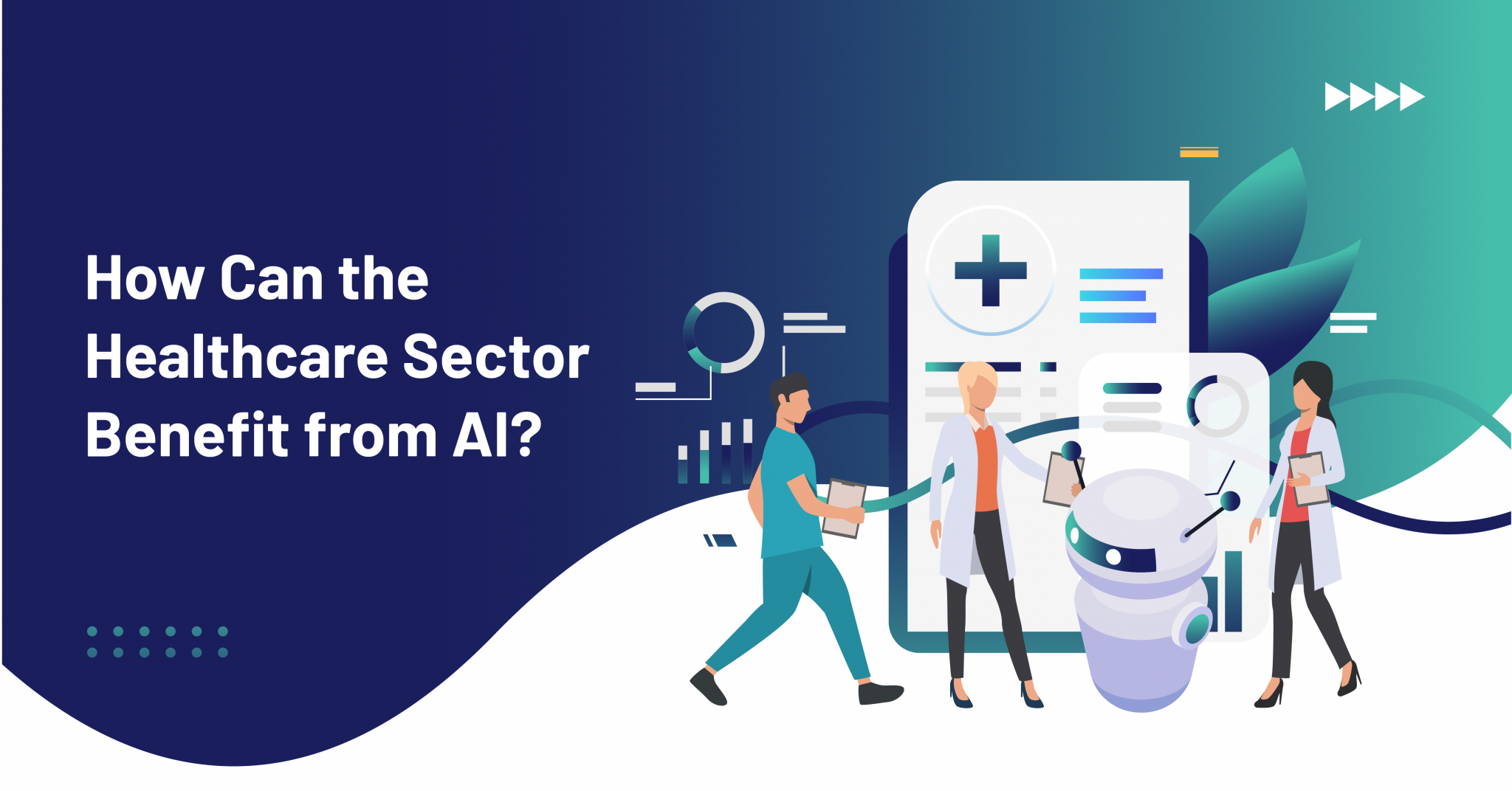
How can the Healthcare Sector Benefit from AI?
Over the last decade, AI (Artificial Intelligence) and Machine Learning (ML) made their mark in several industries worldwide, and it is no different for the healthcare domain. Furthermore, the healthcare sector is continually evolving, and medical data is becoming exceptionally … Read More