Machine learning (ML), a subset of Artificial Intelligence, is a powerful technology that is packing a punch with mind-blowing innovations to empower modern businesses. The concept of machine learning might sound complex and a bit far-fetched, but the truth is it is being widely used by both small startups and mega-corporations.
For any business, be it a product or service, customer journey mapping is one of the fundamental operations to drive value and ROI (Return on Investment). And now, Machine Learning is shaping the process of customer journey mapping in extraordinary ways.
What is customer journey mapping anyway?
It is a series of steps a customer takes before he buys a product or avails a service – like, doing his research, discovering a company, considering their offerings, then purchasing the product or service, and finally, becoming an advocate for that brand.
As a modern business, it is imperative for you to gain a clear understanding of how today’s machine learning is shaping customer journey mapping and how your business can leverage the best from this powerful technology.
How is machine learning shaping better customer journey mapping?
1) Understanding the user journey from intent, need, and preferences
For a long time, the user journey has been instrumental for business teams in building and maintaining a valuable revenue-generating customer base. They help brands target relevant customer groups, identify their true needs, and provide bespoke solutions to them.
But understanding the user journey through a birds-eye-view will not help. Businesses have to understand the user journey through intent, needs, and preferences. In other words, they have to look into the emotional side of user analytics. Up until now, it was a big challenge to pull it off, but with the aid of machine learning technology, Algorithms are capable of understanding user intent, need, and preference learning through abundant data collected along different touch points of the customers, with the help of this businesses can gain a deeper, keener understanding of consumer mindset.
In order to accomplish this, the ML takes three types of information to understand user intent. So, by and large, user intent, needs, and preferences are three unbreakable links that play a vital role in building a detailed and highly visual journey map. When entrusted this data to machine learning, it can deduce and quickly predict the true needs and preferences of users before they contact businesses, which means, businesses can proactively contact them before they approach other competitors.
Once businesses gain a clear understanding of user journey through intent, needs, and preferences, they can start deploying a suitable machine learning framework to build a meaningful customer persona.
2) Building a customer persona using Machine Learning Frameworks
Personas are fictional characters, which are heavily based on real customers. Businesses can now internalize the personas to have a clear focus on their work. They can now refer to users by their name, considering how each product feature or machine learning capability they develop will actually impact their customers personally.
Machine learning, by analyzing huge volumes of data, creates clusters of personas with names, hobbies, and other lifestyle tidbits to bring them to life. It also narrates their objectives and goals relating to the product thus building a complete and credible customer persona.
A good machine learning algorithm blends domain expertise and expert intuition. It means that machine learning models must be designed with team members who have a deep understanding of three things: the business domain, the familiarity of data sets, and a deep intuition of customers true needs.
Machine learning clustering algorithms are used to identify the patterns from the customer data (from different sources) and then seamlessly classify customer behavior to a matching persona in real-time.
There are many ML algorithms businesses can use to offer a personalized experience to their end customers. Of all the algorithms available, there are two most distinguished algorithms that are universally trusted and widely used. They are: Supervised and Unsupervised.
While both these types of machine learning are crucial in creating predictive analytics and customer journey mapping, they are used in different situations and for different datasets. However, the key difference between the two is what you want and what you do not want your ML model to predict.
Let us see how these two ML models are impacting customer journey mapping.
How supervised and unsupervised ML impact customer journey mapping
Though there are many ML algorithms, the two most distinguished and widely deployed algorithms are: Supervised and Unsupervised. While both these types of machine learning are crucial to predictive analytics and customer journey mapping, they are used in different situations and for different datasets. However, the key difference between the two is what you want and what you do not want your model to predict.
Supervised Algorithm:
In this method, the system uses the examples used in the past to get the desired output. In other words, the model is trained to predict the results by using an old set of inputs. After training, the model compares the new results to the old ones and automatically and accordingly updates their data to improve the prediction pattern.
Unsupervised Algorithm:
In this method, experts do not use old data to train the model. In other words, there is no “supervisor” to provide the model with previous examples,. Hence the name unsupervised algorithm. The model is designed to learn by itself and predict the output based on its own observations.
Both supervised and unsupervised impact customer journey mapping in their own respective ways. But by understanding the true significance of each type of business can use them to their own advantage to improve customer journey mapping. For example, supervised machine learning works in two ways- classification and regression. In classification, the model can clearly predict which groups your data falls into—loyal customers and customers who are likely to churn. in regression, for example, the model can predict how long a mechanical part in a factory will last before it needs replacement.
These granular level methodologies in ML frameworks can help businesses to efficiently and quickly improve customer journey mapping, which the agents can seamlessly use to deliver a personalized experience for their target users, eventually leading to improved business growth and increased revenue for an organization.
Once an impactful customer journey mapping and persona building in place, it’s time to set it a motion to create personalized customer experiences.
Creating personalized customer experiences
A meaningful customer journey map reflects customers actual experiences. By fusing persona and customer journey mapping data with an appropriate machine learning algorithm, one can predict customer behavior in real-time and rapidly create personalized suggestions or recommendations for every individual customer.
Let’s see how customer journey analytics help marketing teams in delivering personalized customer experiences for both new and loyal customers.
- With customer journey analytics into their fold, the marketing team can not only identify the most important customer journeys but also can automatically orchestrate actions for each customer at the right time, through their preferred channel, based on their entire experience.
- By setting up triggers along the journey, the team can activate real-time recommendation, content suggestion, dynamic offering their target customer group. These real-time triggers are a lot easier to manage and are more effective than the traditional rule-based, coded systems of the past.
- By integrating machine learning-powered customer journey analytics with its existing marketing tech stack, the team can maximize user engagement and optimize user experience with real-time, personalized messages.
The upshot of all is that businesses can take their marketing performances and customer experience campaigns to a whole new level achieving significant business and overall improved customer satisfaction.
Conclusion
As more and more customers today are expecting personalized, relevant information-driven by their preferences, more and more companies are moving over traditional marketing methods and are employing AI and ML-based personalization solutions for better customer engagement. evolving algorithms and cognitive abilities are empowering businesses to achieve the highest impact on customer experience and overall business growth.
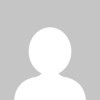
Leave a Reply