Thanks to chatbots and personal assistants, Natural Language Processing (NLP) – an AI-based technology, has become an imperative part of our daily lives. People may have just heard of the term, but few understand the complexities of NLP algorithms and how we use them every day.
You can place NLP solutions into three comprehensive categories related to machine learning, text analytics applications, etc.
1. OCR (Optical Character Recognition) and Language Processing
NLP algorithms can enhance the effectiveness of your text data and can convert scanned documents like invoices, tickets, agreements, etc., into searchable text files for greater efficiency.
2. An Understanding of Natural Language
Using NLP technology, you can:
- Use deep learning to encode phrases and words.
- Extract metadata from text like keywords, syntax, emotions, etc.
- Classify documents, infer author characteristics, and excerpt relevant facts.
- Generate predictive machine learning models.
3. Generating Natural Language
Humans don’t use language to analyse the structure of a given data. Instead, we all use language to communicate and analyse purpose. NLP generates and reflects information through narratives, using deep learning models to define, summarise or elucidate data like humans.
Natural Language Processing Capabilities in the Aviation Sector
1. Classification of documents
Each airline deals with a considerable amount of documentation, for example, instructions, agreements or contracts. NLP solutions using OCR, computer vision, delineating document categories and keyword analysis can rapidly scan all the data and provide insights to decision-makers.
2. Maintenance of the aircraft
NLP helps identify potential repeat defects of the aircraft. This is mainly based on RDM – Recurring Defects Management. It uses metadata based on serial numbers, component descriptions and part numbers to automatically discover the connection between the potential recurring defect to the exact component and how it can affect the aircraft.
3. Search geological data
NLP uses inverse image search and linguistic AI capabilities to help the aviation sector retrieve all relevant information about several geographical locations and places worldwide using text analytics and processing functions. This can be done in seconds.
4. Drone aircraft control system
Using voice recognition and a structured yet intuitive language for air traffic control, NLP helps build an independent planning system that enables the monitoring of the control of the unmanned aircraft and the aviation traffic controller domain.
5. Analysis of flight safety report
Information extraction through NLP solutions can help users identify hidden trends and analyse aviation safety reports. It further helps classify safety-related documents that provide an interactive search tool to extract this information.
Applying predictive analytics to ensure and supervise flight safety and aircraft maintenance can prevent hefty expenses for delays and cancellations. Airlines use predictive maintenance to retrieve and control sensors that monitor aircraft health. It helps generate notifications, alerts and aviation safety reports so employees can identify potential malfunction issues and proactively replace parts. Managers and team leaders can then receive up-to-date information on maintenance operations and component inventory data.
6. Quantification of customer interactions
Sentiment analysis using customer service interactions across call centres, social media properties, research and other unstructured text sources is one of the key tasks performed using NLP in aviation. You can also quantify the effect of negative interactions by incorporating lifetime value metrics.
7. Revenue management (RM)
Revenue management specialists use AI to define destinations, find competent distribution platforms, accommodate prices in specific markets, manage seats and keep airlines competitive and customer friendly at the same time. It helps in validating and monitoring:
- Popular flight routes
- Expected Marginal Seat Revenue (EMSR)
- Ancillary cost optimisation
8. Feedback analysis
Taking a flight and reaching your destination has several touchpoints in-between, like check-in of baggage, in-airport services, in-flight services, searching for gates, the hospitality of the crew, etc. By using AI for feedback analysis, airlines can make informed decisions and meet customer expectations.
9. Messaging automation
The speed and urgency to communicate with travellers is a top priority for airlines. Whether it is about a flight delay, the last call from a passenger, or lost baggage, AI helps communicate a timely response or problem description and respond to customer inquiries. It hence simplifies the workflow of customer service personnel by using algorithms to process natural language or unstructured text.
10. Onboard assistance
Airlines use AI systems with built-in machine learning algorithms to learn about aircraft type and weight, routes, altitude and weather. Based on the insights from the data, the system estimates the optimal amount of fuel required for the flight. Supply management experts also use AI to define the number of snacks and drinks that must be kept on board without wasting them.
11. Anomaly detection
The aviation sector is engulfed in several fraudulent schemes, such as claiming a refund for “lost” luggage, paying with a stolen card, and setting up a fake online travel agency. With the help of AI and aviation NLP, fraud management techniques have become sophisticated that have improved customer authentication and payment security. Airlines have implemented advanced ML-based text analytics software to detect fraudulent transactions and key approaches to fraud management.
12. Self-service at the airport
There is a rise in non-contact technologies, for example, self-service check-in kiosk systems that help automate passengers’ movements and end-to-end multi-terminal solutions that facilitate passenger flow from check-in to boarding. Today, airlines use intelligent systems and biometric technology to recognise passengers with security checks. Non-contact baggage handling reduces passenger check-in time and enhances the customer experience.
About NLP Solutions and Text Recognition
It is true that 80% of the data, in the form of text documents, emails, research sheets, invoices, etc., that companies collect is unstructured data. Using AI and machine learning, airlines will be able to solve various challenges using this unstructured data through:
- Data extraction – Extract text from images and sounds
- Data conversion
- Voice extraction
- Text extraction
- Prototyping and validation of machine learning algorithms using transformed text data
- Classification and contextual search
- A scalable architecture that supports real-time search queries and data transformation
- Cloud, parallel processing, and network settings
Statistics Regarding Adoption of AI
- 80% of all corporate data is in unstructured text files. The extraction of survey results has become much easier because of NLP.
- Several people use Google Translate every day to decipher 100 world languages.
- 47% of companies say they embed at least one AI ability in their business.
- 1 exabyte of data is created on the Int
ernet every day, and this figure is expected to rise, considering the increase in IoT apps. - 85% of customer interaction predictions are managed without the intervention of humans.
Conclusion
The rise of NLP in aviation and intelligent word processing is evident and crucial in this sector. NLP uses machine learning algorithms to aggregate structured and unstructured data for analysis. It helps to extract relevant information for real-time output and support real-time queries. Aviation NLP brings dynamic software development and expertise to deep learning development and builds custom AI voice processing systems that save millions of dollars in flight operations.
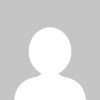
Leave a Reply