The use of digital technologies has skyrocketed due to the pandemic as people are staying at home more than ever. Among various sectors, the media sector has witnessed a steep rise in content consumption on digital platforms. While the increase in video, text and audio content of sports and news is apparent, according to a report, India is the fastest-growing OTT streaming market globally, and it is projected to grow at a CAGR of 28.6% over the next four years. However, such rapid growth in viewership has exposed media providers to ignorant of rich metadata potential in the digital world.
Mostly, media providers rely on a manual process for content creation, hosting, and personalization for their users. This led to a bitter user experience for most people who started binge consuming digital content like movies and news, as the dated practices failed to match user expectations.
In the digital world, media companies that leverage rich metadata gain an edge in the highly competitive market created by the pandemic. With effective rich metadata planning through machine learning, companies can expedite the entire content hosting and personalization for users to enhance user engagement on their platforms.
How Rich Metadata Differs from Metadata?
Metadata is data that describes other data. In other words, metadata can describe your content into various categories. However, rich metadata can further correlate your content into a wide range of tags, providing crucial information about content that can be used to understand users’ interests.
For instance, if you are an OTT content provider and want to host a movie named Men In Black, its metadata would be limited to genres, language, cast, and crew. Obtaining only a few details about content limits the correlation with other topics. But with rich metadata generated by machine learning, for Men In Black, you can get tags like alien, illegal immigration, government agency, criminal, political drama, corruption, among others.
Going a step further with rich metadata can help you connect the dots with other content for hyper-personalization and increase user engagement. While providing bespoke content can keep users on the platform for a more extended period, rich metadata can also automate content management to reduce resources’ cost by eliminating manual work.
How to Automate Rich Metadata Generation and Content Management?
Today, media companies deal with a colossal amount of content; manually creating metadata becomes a tedious job. In media companies, professionals add metadata for every content by either embracing the dated approach of searching on the web or intuition, consuming more time. If obtaining metadata alone takes endless time, imagine how much resources would you spend to generate rich metadata for new content. It would be near impossible to create rich metadata with human efforts.
To assist companies with rich metadata generation, several machine learning-based service providers have developed solutions that can streamline the process of generating rich metadata. Embracing state-of-the-art solutions can not only assist you in quickly creating rich metadata but also automate content management.
Media companies hire catalog managers who oversee the content hosting on their platforms. Such methodologies are prone to errors and consume a lot of human resources. But with machine learning, media companies can automatically label rich metadata with the right content and host on the platforms to enhance efficiency and productivity.
Rich Metadata for Personalization and Content Creation
After content creation and management, media providers’ next vital aspect is to increase user engagement with continuous relevant content. If a user interacts with content, you can collect several rich metadata of the content to understand users’ interests. More information with rich metadata allows media firms to understand the context of user behavior, thereby allowing machine learning models to assimilate them better for targeting with potential exciting content. The more data organizations have about the type of content users interact with, the better the profiling capabilities. If media firms can create exceptional users’ profiles, they can increase engagement with accurate targeting.
Another approach to improve engagement on media platforms, along with personalization, is to create content that your users like the most. When users engage with content, the generated rich metadata can be used to understand popular tags associated with the content. With such insights, you can create content that includes those popular tags and continue delivering content that users like the most for increasing engagement.
Machine Learning Techniques for Personalization
When it comes to personalized content for users for increasing engagement, various machine learning techniques like recommender systems are leveraged based on the type of content.
Recommender systems in media are broadly divided into two sections: content-based and collaborative. Content-based algorithms recommend content that is similar to the rich metadata of already consumed media. One of the popularly used methodologies in content-based technique is ‘cosine similarity,’ where profile and item vectors are created to assess the relationship; if the angle of cosine is zero, the content is relevant.
Other similar techniques used for content-based recommendation are Pearson’s correlation and Euclidean distance. While Pearson’s approach is used for finding similarity within a single category of content, the Euclidean distance method finds the closest distance in n-dimensional space among content for relevancy.
While content-based recommendation relies more on rich metadata of content, collaborative filtering extensively places weightage on user interaction. Users’ historical engagement with content can be used to classify the type of content a user may like. Besides, collaborative filtering can be carried out by comparing several users’ interests to find relevant content.
However, the implementation of different machine learning methods can not deliver the desired results until the models are monitored for enhancing efficiency. After forecasting and targeting users with relevant content, it has to be observed whether a user engaged with the content machine learning models suggested them based on similarity metrics. For this, metrics like root mean square error, mean reciprocal rank, recall, precision, and more are used to optimize the machine learning models for enhancing accuracy.
Integrating State-of-the-art Machine Learning Models in Media Organizations
Equipping superior machine learning models requires a team of experts who can simplify the entire process, right from collecting users’ data to building machine learning models to monitoring them and complying with privacy regulations. One such company is RecoSense, an end-to-end AI services provider, which helps media organizations increase user engagement by integrating bespoke machine learning models.
Leveraging rich metadata can not only cut costs but increase user engagement by multiple folds, provided media companies can deploy machine learning models that can completely automate their operations. In order to tap into the rising market of media consumption, organizations should leverage rich metadata for automation and customization to increase business growth.
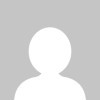
Leave a Reply